Welcome to the DSI Newsletter
Our newsletter is a compendium of breaking news, the latest research, outreach efforts, and more.
Register for Virtual WiDS Livermore on March 7
The annual Women in Data Science (WiDS) conference returns on Monday, March 7, which is International Women’s Day. LLNL will again host a regional event in conjunction with the worldwide conference. The all-day WiDS Livermore event will be entirely virtual and free. Everyone is welcome to attend. Registration is open until February 27.
Sponsored by the DSI and LLNL’s Office of Strategic Diversity and Inclusion Programs, WiDS Livermore will include a livestream of the Stanford conference and networking opportunities. Returning this year is the popular “speed mentoring” session, where mentees...

Happy New Year from the DSI Council
The start of a new year is an exciting time because of the opportunity to appraise our data science community’s myriad accomplishments as well as preview upcoming projects and events. Like other areas of LLNL, the DSI has adapted to evolving pandemic restrictions and workplace policies to prioritize safety.
We were pleased to sponsor and contribute to multiple activities in modified or virtual formats: our monthly seminar series, the fourth annual Women in Data Science (WiDS) Livermore event, a new career panel series inspired by WiDS, the Machine Learning for Industry Forum (ML4I), and a...
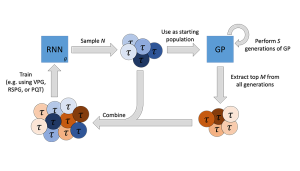
Five Papers Accepted to NeurIPS 2021
The annual Conference on Neural Information Processing Systems (NeurIPS) returns December 6–14. LLNL work has been accepted at the prestigious machine learning conference in past years; in 2021 researchers have five accepted papers. Preprints are linked here.
- A Winning Hand: Compressing Deep Networks Can Improve Out-of-Distribution Robustness – James Diffenderfer, Brian Bartoldson, Shreya Chaganti, Jize Zhang, and Bhavya Kailkhura
- Designing Counterfactual Generators using Deep Model Inversion – Jayaraman Thiagarajan and colleagues from Arizona State University, IBM Research, and Stanford...

Counterfactual Generators for Deep Models
LLNL’s research into machine learning (ML) interpretability continues with an investigation of counterfactual explanations—those that synthesize a hypothetical result based on small, interpretable changes to a given query image. Existing approaches rely extensively on pre-trained generative models or access to training data to create plausible counterfactuals that support users’ hypotheses.
LLNL’s Jayaraman Thiagarajan and colleagues from Arizona State University, Stanford University, and IBM Research have developed a technique called DISC—Deep Inversion for Synthesizing Counterfactuals—that...

4D Computed Tomography Reconstructions
Computed tomography (CT) is a type of x-ray imaging technology with a range of applications for clinical diagnosis, non-destructive evaluation in industry, baggage inspection, and cargo screening. CT scanners capture a sequence of angles around an object. Reconstruction algorithms then estimate the scene from these measured images. 2D and 3D CT imaging of static objects are well-studied problems with theoretical and practical algorithms. However, reconstruction of scene changes and measurements over time, known as dynamic 4D CT, can yield spatiotemporal ambiguities. (Image at left: 4D CT of...

New Bayesian ML Code Released
A new Bayesian machine learning (ML) code, MuyGPyS (pronounced my-jee-pies) has been developed as part of a Laboratory Directed Research and Development Strategic Initiative to address needs for native uncertainty quantification in ML predictions, learning with bounded training times, support for combining ML and model-based Bayesian inference frameworks, and extending the data sizes allowable in Gaussian process (GP) models.
The MuyGPyS code and algorithm offer best-in-class performance on community GP regression benchmarks, as well as image classification performance competitive with or...
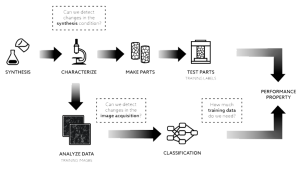
Deep Learning for Materials Discovery
Deep Learning (DL) models are proving useful for a number of materials science applications including materials discovery, microstructure analysis, and property predictions. In a recent paper in ACS Omega, LLNL researchers propose a unified framework that leverages the predictive uncertainty from deep neural networks to answer challenging questions materials scientists usually encounter in machine learning (ML)–based material application workflows.
Specifically, the team demonstrates that predictive uncertainty from uncertainty-aware DL approaches (particularly deep ensembles) can be used to...
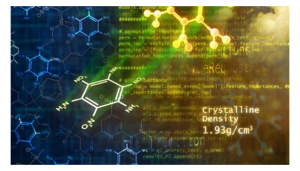
Research in Feedstock Optimization
A long-held goal by chemists across many industries, including energy, pharmaceutics, energetics, food additives, and organic semiconductors, is to imagine the chemical structure of a new molecule and predict how it will function for a desired application. In practice, this vision is difficult to realize, often requiring extensive laboratory works to be able to synthesize, isolate, purify, and characterize newly designed molecules to obtain the desired information.
A team of LLNL materials and computer scientists have brought this vision to fruition for energetic molecules by creating machine...
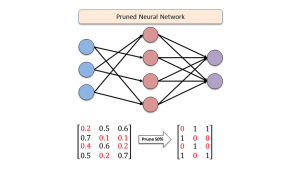
New Research in Machine Learning Robustness
LLNL postdoctoral researcher James Diffenderfer and computer scientist Bhavya Kailkhura are co-authors on a paper that offers a novel and unconventional way to train deep neural networks (DNNs). The LLNL team shows both empirically and theoretically that it is possible to learn highly accurate NNs simply by compressing (i.e., pruning and binarizing) randomized NNs without ever updating the weights. This is in sharp contrast to prevailing weight-training paradigm—i.e., iteratively learning the values of the weights by stochastic gradient descent. In this process, Diffenderfer and Kailkhura...
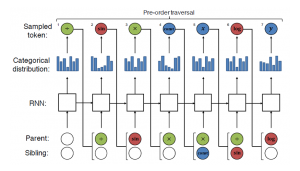
Spotlight: New Research Ranked Among Top AI Papers
Symbolic regression is the ML task of discovering tractable mathematical expressions to fit a dataset, yet the AI community has not fully explored deep learning approaches that explore this challenging space. In a paper accepted as an Oral Presentation at the upcoming International Conference on Learning Representations (ICLR), an LLNL research team proposes a framework that leverages deep reinforcement learning for symbolic regression via a simple idea—use a large model (neural network) to search the space of small models (expressions). With an Oral acceptance rate of only 1.5%, the team’s...

Spotlight: Research Team Recognized for COVID-19 Model
A machine learning model developed by a team of LLNL scientists to aid in COVID-19 drug discovery efforts was a finalist for the Gordon Bell Special Prize for High Performance Computing-Based COVID-19 Research. Using the Sierra supercomputer, the team created a more accurate and efficient generative model to enable COVID-19 researchers to produce novel compounds that could possibly treat the disease.
The team trained the model on an unprecedented 1.6 billion small molecule compounds and 1 million additional promising compounds for COVID-19, which reduced the model training time from 1 day to...

Spotlight: Special Recognition for Researchers
Since joining LLNL as a postdoc in 2013, Jayaraman Thiagarajan’s research has grown to include multiple related fields. This exploration ranges from deep learning–based graph analysis to machine learning (ML) and artificial intelligence (AI) solutions for computer vision, healthcare, language modeling, and scientific applications. Thiagarajan recently received an LLNL Director’s Early Career Recognition Award for his authoritative work and key contributions. He earned a PhD in Electrical Engineering from Arizona State University.
Peer-Timo Bremer has accepted the role as LLNL’s Point of...
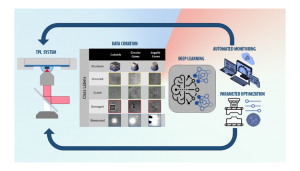
3D Printing Meets Machine Learning
Two-photon lithography (TPL)—a widely used 3D nanoprinting technique that uses laser light to create 3D objects—has shown promise in research applications but has yet to achieve widespread industry acceptance due to limitations on large-scale part production and time-intensive setup.
LLNL scientists and collaborators are using machine learning (ML) to address two key barriers to industrialization of TPL: monitoring of part quality during printing and determining the right light dosage for a given material. The team developed an ML algorithm trained on thousands of video images of TPL builds...
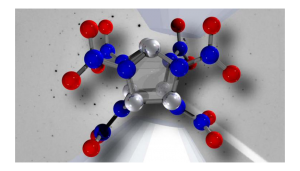
Spotlight: Mentoring the Next Generation
For the second year in a row, the DSI teamed up with the University of California at Merced to offer a two-week Data Science Challenge at the beginning of June. The intensive program provided mentors, assignments, virtual tours, and seminars. Under the direction of LLNL’s Marisol Gamboa and UC Merced’s Suzanne Sindi, 21 students worked from their homes through video conferencing and chat programs to develop machine learning (ML) models capable of differentiating potentially explosive materials from other types of molecules.
The UC Merced students were divided into five teams, each led by a...

Spotlight: Materials Science Meets AI
LLNL scientists have taken a step forward in the design of future materials with improved performance by analyzing its microstructure using AI. The work recently appeared in the journal Computational Materials Science.
Technological progress in materials science applications spanning electronic, biomedical, alternate energy, electrolyte, catalyst design, and beyond is often hindered by a lack of understanding of complex relationships between the underlying material microstructure and device performance. But AI-driven data analytics provide opportunities that can accelerate materials design...