The DSI recognizes contributions staff make to LLNL's data science community through periodic Data Scientist Spotlights.
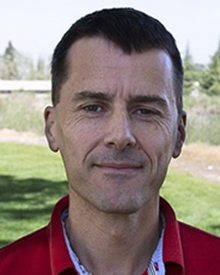
Nicolas Schunck
Staff Scientist
Nicolas Schunck, a staff scientist with the nuclear data and theory group in LLNL’s Physical and Life Sciences Principal Directorate, researches computational nuclear theory with a particular focus on nuclear fission. Schunck develops models and simulations that predict the properties of short-lived radioactive species in nucleosynthesis mechanisms. He’s also working on a predictive theory of nuclear fission for stockpile stewardship and nuclear forensics programs. Schunck was educated at the University of Strasbourg and worked as a postdoc in the UK, Spain, and at Oak Ridge National Lab before coming to Livermore 13 years ago. A prolific communicator, he lists 66 papers as author or coauthor and edited the textbook Energy Density Functional Methods in Atomic Nuclei. He’s helping mentor five postdocs and always looks forward to working with postdocs and summer interns. “Teaching forces me to question what I know and what I do on a regular basis, which is refreshing,” says Schunck. “Constant reassessment is critical to staying at the cutting edge of research.” Schunck finds his data science work provides valuable perspectives on problems in nuclear science, stating, “By looking at the same old problem in a completely different light, we can come up with innovative solutions.”

Aisha Dantuluri
Staff Scientist
Aisha Dantuluri is a staff data scientist in LLNL’s Applications, Simulations, and Quality division. Her projects mainly contribute to the Lab’s Weapon Physics and Design program. She uses machine learning (ML) for image processing to make better quantitative use of simulated and experimental radiographic data for stockpile certification. She also works on automating time series analysis in the Diagnostics Development Group, which involves jump off detection in photo Doppler velocimetry data. Data science inspires Dantuluri due to its collaborative nature and range of applications. “Everybody is open to sharing knowledge, and I think that makes data science a fun thing to work on. You can pick any problem in any field, and they probably need a data scientist,” she said. Since she began working at LLNL in 2020, Dantuluri has sought to improve the sense of community amongst data scientists in different organizations. She is the current ML working group coordinator for WPD and hopes to foster more involvement with the Strategic Deterrence organization’s data science community in the future. Dantuluri earned a master’s degree in computational science at UC San Diego and a bachelor’s degree in engineering physics at the Indian Institute of Technology, Hyderabad.

Qingkai Kong
Staff Scientist
Qingkai Kong is a staff scientist supporting LLNL’s Geophysics Monitoring Program, Nuclear Threat Reduction, and the Atmospheric, Earth, and Energy Division where he contributes to a variety of machine learning–focused projects. As a part of the Source Physics Experiment project, Kong and colleagues use physics to improve the generalization capabilities of their machine learning model. He is heavily involved in seismology and machine learning through the Laboratory Directed Research and Development Program and the Low-Yield Nuclear Monitoring project, as well as carbon storage research through the SMART (Science-informed Machine Learning for Accelerating Real Time Decisions in Subsurface Applications) project. Kong enjoys the constant learning opportunities that his work offers, stating, “Combining decades of physics knowledge with recently developed deep learning methods is both an exciting and challenging area.” He is also an active LLNL community member—mentoring summer interns, speaking at an annual meeting of the Seismological Society of America, leading the Machine Learning in Seismology discussion group—and coaches a local children’s soccer team. Prior to joining the Lab 2021, Kong was a data science researcher at UC Berkeley and a researcher in Google’s visiting faculty program. He earned his PhD in geophysics from UC Berkeley.
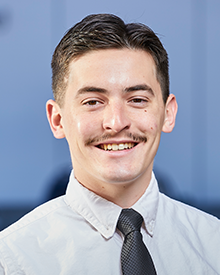
Robert Cerda
Research Support Engineer
Research support engineer Robert Cerda is new to the Lab, but as a graduate of UC Berkeley’s Air Force ROTC program and a newly commissioned second lieutenant in the United States Space Force, he’s no stranger to an ambitious challenge. Cerda joined LLNL as an intern in May of 2022, transitioned to staff after graduation, picked up his commission in May 2023, and is now automating polymer-related projects with the Materials Engineering Division. His current focus is the automation of database pipelines, postprocessing scripts, and physical processes through software. He recently presented a poster at the Artificial Intelligence for Robust Engineering & Science Conference at Oak Ridge National Laboratory on direct-ink write automation and database-pipeline refinement for leveraging big data. “Basically, I do all things computer and data science as they relate to additive manufacturing,” says Cerda. He enjoys helping interns from his ROTC/MARA program and welcomes opportunities to mentor. “I feel it’s especially important for me to impart what I’ve learned to those who are where I was not long ago.” He also enjoys working at the Lab and flexing his creativity to solve a problem. “I like working on cutting-edge projects of national importance,” says Cerda. “I also just think working at the Lab is fun.”
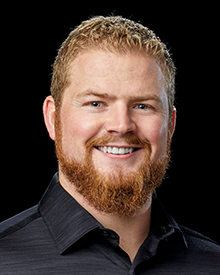
Mason Sage
Data Science Engineer
Mason Sage’s zeal for interdisciplinary research was ignited in trade school, when he discovered mechatronics—a hands-on fusion of electrical engineering, mechanical engineering, and computer science. He went on to an Engineering/Computer Science degree, a robotics engineering stint at Tesla, and work in the semiconductor field before gravitating to LLNL’s high-stakes challenges in 2022. Sage is a staff research engineer supporting the Materials Engineering Division where he’s helping to build a system for the Modular Autonomous Research Systems (MARS) project that’s automated enough to perform specified processes and intelligent enough to make decisions based on experience. For the HAMMER project, he builds mechatronics elements. He especially likes designing processes for generating data and then finding automated processes to refine those processes, stating, “Automating projects opens up a lot of doors.” Sage has enjoyed success in his short time at the Lab and looks forward to applying his expertise to concepts for self-driving laboratories. “I like working on whatever is cutting edge and staying ahead of the curve,” he says. “Working in national security has been rewarding.”
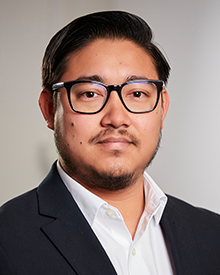
Aldair Gongora
Staff Scientist
Aldair Gongora is a newly minted staff scientist, having transitioned from postdoc status on October 1st. As a member of the Analytics for Advanced Manufacturing group, he supports the Advanced Manufacturing Lab (AML), the Center for Engineered Materials and Manufacturing (CEMM), and the DSI. Gongora is working on three projects in data science and machine learning. First, he’s accelerating the design of additive manufacturing applications by combining machine learning and automated experimentation to select and conduct experiments without human intervention (“self-driving” labs). His other projects are focused on scaling up technologies in climate and energy applications, and developing and using a modular autonomous research system (MARS) for biological applications. For both, he designs intelligent, adaptive frameworks to connect various information sources—e.g., chemical and materials data, device-level performance, technoeconomic-analysis predictions—across scales. Born in Belize, Gongora won a scholarship to Rockhurst University (Kansas City) for his bachelor’s degree, following up at Boston University with a PhD in autonomous experimentation for mechanical design. He recently presented his research at an MIT autonomous research seminar and an American Physical Society meeting. Gongora’s journey has taken him far from home, and he’s grateful for the support along the way. “I feel fortunate to have had conversations with many great people who inspired and mentored me during my education and here at LLNL,” he says.
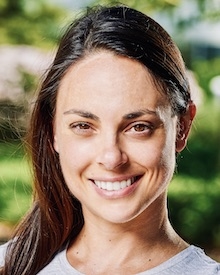
Giselle Fernández
Data Scientist
Data scientist Giselle Fernández contributes to an “exhilarating and immensely rewarding” breadth of LLNL research. She serves as machine learning (ML) lead for projects involving fusion energy design optimization, material deformation, and post-detonation flow transport. “Machines undoubtedly will play a pivotal role in our future,” she says. “My deep involvement in data science makes me feel connected to that future in an unprecedented way.” After completing an aerospace engineering PhD at the University of Florida and postdoctoral research at Los Alamos National Lab, Fernández came to Livermore in 2020, joining the Atmospheric, Earth, and Energy Division to apply expertise in ML techniques and uncertainty quantification to research utilizing high-performance computing resources. “Being part of the LLNL community affords me the honor of working for the safety and security of our nation—a responsibility I take immense pride in.” Dedicated to outreach and mentorship, Fernández regularly presents workshops and tutorials, authors news articles, and leads interdisciplinary ML discussions. She also hosts students every summer at Livermore. “My mom, who devoted her career to teaching early on, always fascinated me with her passion for education. Now, hearing students say, ‘I learned so much from you,’ I appreciate how deeply rewarding it is.”
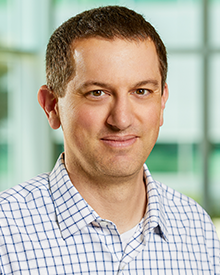
Andrew Gillette
Computational Mathematician
Andrew Gillette’s mathematics career has evolved from pure theory to applied math, and now it also includes machine learning, cognitive simulation, and other data science techniques. The intersection of these fields poses interesting questions and unique possibilities. He states, “Large numerical datasets appear all over the Lab and in all kinds of sciences, so one challenge is figuring out the right approach for modeling the data. How much data do you need, and is the data you already collected enough?” With projects ranging from implicit neural representations in 3D visualization to artificial intelligence in space science, Gillette says a mathematical perspective is necessary in data-driven problems and among LLNL’s multidisciplinary teams. This summer he is mentoring a student who is using reinforcement learning to guide adaptive mesh refinement. “Whether it’s through publications, the code I write, or people I mentor, my work at the Lab will have an impact,” he notes. Gillette holds a PhD in Mathematics from the University of Texas at Austin. He was a tenured professor at the University of Arizona before joining LLNL in 2019.

Jiachen Yang
Machine Learning Researcher
Since joining the Lab’s Computational Engineering Division in 2022, Jiachen Yang has been developing machine learning (ML) methods that speed up computational antibody design for rapid response against new pathogens. He is excited about this intersection of ML and science, stating, “New ML techniques accelerate science by unlocking the latent information of large scientific datasets and simulations, while science provides new challenges such as interpretability and symmetry constraints that drive ML advances.” Yang also develops new deep reinforcement learning algorithms for adaptive mesh refinement, as described in papers accepted to the 2023 AISTATS and AAMAS conferences. He enjoys working on unsolved problems and creating new approaches for old problems—enthusiasm he will impart while mentoring a Data Science Summer Institute intern this year. “Having worked at multiple industry research labs during my graduate studies, I find that internships are valuable for sparking new ideas and research directions, and I find value in creating such opportunities for others,” says Yang. He holds a PhD in Machine Learning from Georgia Tech and is part of LLNL’s award-winning deep symbolic optimization team.
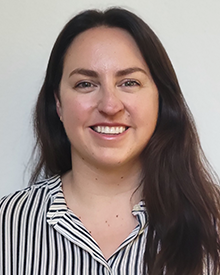
Rebecca Rodd
Data Specialist
A crucial aspect of data science—particularly at LLNL and across the Department of Energy (DOE)—is the management of big data across different domain expertise and programs. Since joining the Lab’s geophysical monitoring programs in 2019, Rebecca Rodd has focused on data cleaning and ingestion, developing geophysical data management standards, and building data infrastructure for several of NNSA’s Defense Nuclear Nonproliferation R&D projects. “Over the last decade, innovation in data management techniques and tools has led to improvements in data storage, rapid data transfer, integrated data management systems, cloud and hybrid computing, and other areas,” she explains. “The DOE has many data management successes, and applying them to geophysical programs and datasets is exciting and challenging due to the multi-laboratory and multi-phenomenology requirements, solving multi-lab access control and varied security policies, and inconsistent metadata standards across and within domain areas.” Rodd thrives on learning new technologies and in 2022 assumed leadership of the annual DOE Data Days workshop (D3), which brings together data management practitioners, researchers, and project managers to promote data management for higher quality, more efficient R&D across the DOE complex. She notes, “Data management often does not get as much attention at data-focused DOE meetings, so D3 offers a place for more collaboration in this area.” Rodd holds a B.S. in Geology from UC Davis and an M.S. in Geosciences from the University of North Carolina at Chapel Hill.
Priyadip Ray
Research Scientist
Priyadip Ray came to LLNL out of a desire to do impactful work. Growing up in India, Ray was inspired by his father, a physicist, to pursue science and discovered the potential that engineering had to change lives. After finishing his undergraduate and graduate work in India, Ray obtained his PhD in electrical and computer engineering from Syracuse University and completed a stint as a postdoc at Duke University before joining the Lab. At LLNL, Ray applies ML and AI to clinical data and electronic health records to create predictive models of diseases including amyotrophic lateral sclerosis, sepsis, and COVID-19. Through these improved models, clinicians could potentially uncover novel therapeutics or detect signatures and diagnose diseases much earlier than they are currently able to, providing more lead time to develop countermeasures and prepare for future pandemics. Ray enjoys engineering because it allows him to work on “Big Science” and multidisciplinary projects and said he has “never found a better set of colleagues anywhere else.” He encourages young engineers to seek out a specialized niche and get involved in research projects through internships, at companies or at universities to find the best fit. “Every research group needs diverse people, because everybody brings some unique strengths, and that contributes a lot. My advice would be to reach out and get involved in projects, even if they’re not directly in your area, but that give you that bigger picture view that will really help you succeed.” Watch him discuss his background and projects (2:15).
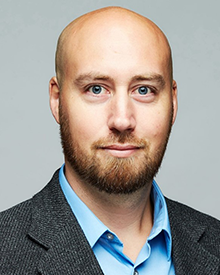
Ruben Glatt
Senior Staff Researcher
Working at LLNL gives Ruben Glatt, a senior staff researcher in machine learning, the opportunity to solve globally important problems. He currently leads a Laboratory Directed Research and Development feasibility study using reinforcement learning (RL) to investigate energy-efficient transportation and, in other projects, applies generative models and domain concepts to improve trust in model predictions. “Keeping up with the developments in the fields of data science and machine learning can pose a challenge, yet the recent progress made in these areas holds immense potential to revolutionize energy generation, healthcare, and other industries,” he explains. “As we embark on a new era where artificial intelligence holds the power to master an increasing number of processes, it is my aspiration to ensure that research remains aligned with humanistic values, so that we can not only fully realize the benefits of these advancements, but also minimize any potential existential risks.” In 2022, Glatt chaired the Lab’s Center for Advanced Signal and Image Sciences (CASIS) workshop and was part of the research team whose deep symbolic optimization method won the first-ever worldwide symbolic regression competition. He has presented his research at numerous venues including premier machine learning conferences, and contributed a chapter on efficient RL to the Federated and Transfer Learning book, published by Springer in 2022. Glatt joined the Lab in 2019 after completing a PhD in Computer Engineering with a focus on knowledge transfer in RL.
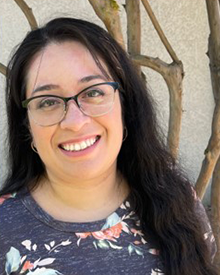
Mary Silva
Data Scientist
With an M.S. in Statistics and Applied Math from UC Santa Cruz, Mary Silva knows firsthand how the Lab’s multidisciplinary approach to teamwork can elevate everyone involved. “Even without an extensive background in biology, I can contribute to vaccine development and target identification while utilizing domain experts’ knowledge to interpret models and results. These experiences have inspired me to take computational biology courses. A data scientist is forever a student,” she explains. A former DSSI intern, Silva joined LLNL in 2020 and today works on active learning and Bayesian spatial models for rapid design of COVID-19 antibodies, as well as enhancing machine learning models through the multi-institutional Scalable Precision Medicine Open Knowledge Engine (SPOKE) project. As a mentor, she helps students improve their weaknesses. For instance, she says, “If a student doesn’t have public speaking confidence, I can give them opportunities to present their work to an audience.” Silva also co-organizes the Lab’s Women in Data Science (WiDS) event and datathon challenge. “I found my Lab internship by attending WiDS Livermore with my professor, and the ability to socialize and network with LLNL researchers kicked off my career,” she states.

Amanda Muyskens
Statistician
According to Amanda Muyskens, the best thing about being a statistician is the opportunity to work on—and learn from—unique challenges. She joined the Lab in 2019 after earning a PhD in Statistics from North Carolina State University, and today her research includes Gaussian processes (GP), computationally efficient statistical methods, uncertainty quantification, and statistical consulting. Muyskens is the principal investigator for the MuyGPs project, which introduces a computationally efficient GP hyperparameter estimation method for large data (watch her DSI virtual seminar and the MuyGPs video). Her team used MuyGPs methods to efficiently classify images of stars and galaxies, and they developed an open-source Python code called MuyGPyS for fast implementation of the GP algorithm. Muyskens credits the team dynamic for its success, noting, “We constantly teach each other from our disciplines and achieve things together that wouldn’t have been possible alone.” In 2022, Data Science Summer Institute (DSSI) students contributed to MuyGPs with parameter estimation optimization and an interactive visualization tool. “Students bring a new perspective to the work, and I’m inspired to see them tackle problems in ways that those of us entrenched in the applications may never have considered,” Muyskens says. Most recently, she assumed DSSI co-directorship and began a collaboration with Auburn University data science students.
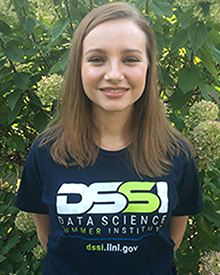
Emilia Grzesiak
Data Scientist
With Biomedical Engineering degrees from Duke University, Emilia Grzesiak contributes to LLNL’s COVID-19 research by comparing simulations to bioassays that measure the binding affinity between the virus’s variants and antibody candidates. She also builds analysis and visualization tools to identify antibody designs that could be useful drug candidates. “I’m excited to help with therapeutic design decision-making and speed up the drug-design process,” she says. Grzesiak joined LLNL’s Global Security Computing Applications Division in 2021 after interning with the Data Science Summer Institute (DSSI) the previous year. Now, as a first-time mentor, she states, “I’m figuring out when to let go of the reins and when to step in more. Establishing trust and open communication is important, as making those judgment calls becomes easier when you understand how your intern approaches problems and what kind of advice they respond best to.” Grzesiak recently shared her career journey and research highlights during a DSI-sponsored panel discussion and a seminar for the DSSI's class of 2022.

Hyojin Kim
Data Scientist and Machine Learning Researcher
Hyojin Kim is a data scientist and machine learning researcher at LLNL’s Center for Applied Scientific Computing. His research interests in machine learning and computer vision are recently related to applications for computed tomography, AI-driven drug discovery, scalable and distributed deep learning, and multimodal image analysis. He also has hands-on experience applying GPU computing to challenging problems in these areas. Balancing research and development, as well as learning domain knowledge, are crucial because, Kim says, “I often see data scientists trying to apply a new technique to a particular domain application where it may not be suitable.” This summer, Kim mentored students from two University of California campuses in DSI’s Data Science Challenge to accelerate drug discovery for COVID-19. During the intensive two-week program, he states, “Many of the students I met were enthusiastic, and some of them came up with brilliant ideas that I never thought about before. Students majoring in fields other than computer science are quite knowledgeable in data science, and I actually feel the growing popularity of data science in recent years.” Kim joined LLNL in 2013 after earning his Ph.D. in Computer Science from UC Davis in 2012.

Kevin McLoughlin
Computational Biologist
Kevin McLoughlin has always been fascinated by the intersection of computing and biology. As a graduate student in the 1980s, he worked on early neural network simulations to understand the human brain. He recalls, “Computational biology as a field didn’t exist then, but that changed when the Human Genome Project launched.” After a stint with a biotech startup, McLoughlin joined the Lab in 2004 to work on pathogen bioinformatics. Since 2017, he has participated in the Accelerating Therapeutics for Opportunities in Medicine (ATOM) consortium, which combines HPC and data science techniques to design drugs for cancer, pathogens, and other diseases. “ATOM is enormously important, draws on my full skill set, and demands that I constantly learn new things. I work with extremely smart people from LLNL and our partners,” he says. McLoughlin helped develop a COVID-19 antiviral drug design pipeline that combines a computational autoencoder framework with machine learning algorithms to propose molecular structures, identify those with desirable properties, and suggest new molecules based on the best results—research that earned one of the Lab’s 2021 Excellence in Publication Awards. He holds a PhD in Biostatistics from UC Berkeley.
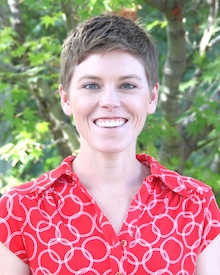
Sarah Mackay
Computational Mathematician
With a PhD in Mathematics from the University of Illinois at Urbana-Champaign, Sarah Mackay enjoys using mathematical techniques to make inferences about real-world systems. She draws on her experience in combinatorial optimization, network science, and statistics to perform risk analyses for LLNL’s Cyber and Infrastructure Resilience program. Mackay designs and implements algorithms to secure infrastructure such as power grids, gas pipelines, and communication systems. “This work involves making assumptions about the structure of the system we’re studying. It can be challenging to know if the assumptions are valid and, thus, if we can trust our conclusions,” she explains. Mackay, who also coordinates the DSI’s virtual seminar series, thrives in the Lab’s culture of interdisciplinary teamwork. She states, “The set of problems one can tackle becomes so much larger when the pool of expertise grows.”
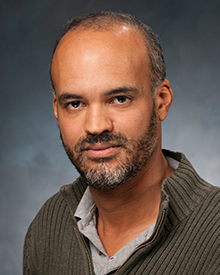
Jonathan Allen
Bioinformatics Researcher
The Lab’s biosecurity mission relies on multidisciplinary expertise in areas such as molecular biology, bioinformatics, high-performance computing, and machine learning. LLNL’s Jonathan Allen seeks to understand biological systems that impact human health and safety, in part by working with the Accelerating Therapeutics for Opportunities in Medicine (ATOM) consortium. “An exciting challenge is to synthesize small molecule compounds proposed by a computational model, physically test them, and revise the model based on the experimental feedback,” he explains. Recently, Allen helped expand LLNL’s partnership with ATOM to include Purdue University. The collaboration gave students an opportunity to apply data science techniques to the drug discovery process, searching for novel therapeutics for cancers and other diseases; future plans include evaluation of new COVID-19 compounds. As a mentor, Allen states, “I hope to contribute to a positive learning environment and encourage a healthy, socially thoughtful research community. Every person develops their skills and interests at their own pace and has the potential to do great things.” Allen joined LLNL in 2007 after earning a PhD in Computer Science and Bioinformatics from Johns Hopkins University.
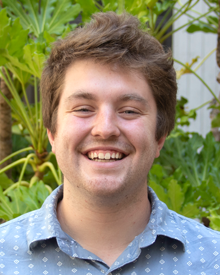
Ryan Dana
Data Scientist
Ryan Dana is a data scientist working in the Global Security Computing Applications Division and the astrophysical analytics group. He was a student intern with LLNL’s Data Science Summer Institute (DSSI) in 2019 and graduated that year with a B.A. in Physics, Astrophysics, and Data Science from UC Berkeley. "The DSSI perfectly connected my coursework in physics, astronomy, statistics, and computer science to solving problems using real-world astronomical data," he says. Dana joined the Lab as a full-time employee in early 2020 and mentored students in the 2021 Data Science Challenge program—a position he returns to in 2022. His research interests include using machine learning techniques to approach astrophysical questions.

Michael Ward
Computer Scientist
Computer scientist Michael Ward strives to improve the world in any way he can. “My motivation is often driven by making things better, whether fixing something that’s broken, providing a better experience for a user, or refining something to be more capable or stable,” he explains. Ward works in LLNL’s Global Security Computing Applications Division on data science projects involving geospatial intelligence, object detection, and imagery processing. “The biggest challenge is keeping up with the pace of the field and supporting technology,” he states, noting that he continually enjoys tackling “tough and unique problems with some of the smartest minds in the field.” Before joining the Lab in 2018, Ward built software for sales training, banking, inventory, and telecommunications. He also taught college-level computer science for four years, and says the experience of finding ways to convey complex ideas and technologies to others has come in handy at the Lab. Ward earned bachelor’s and master’s degrees from the University of South Alabama.
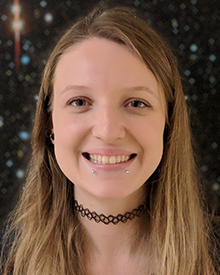
Kerianne Pruett
Astrophysicist
With a passion for outreach and volunteering, Kerianne Pruett enjoys encouraging and inspiring students to pursue STEM careers. She has held roles such as mentor, teacher, and organizer for various K–12 events; led telescope viewings and science demonstrations; and provided resources and support to underrepresented college students, promoting diversity and retention in STEM programs. This summer, Pruett mentored undergraduate and graduate students in two Data Science Challenge sessions—and received awards from LLNL’s National Security Engineering Division and Physical and Life Sciences Directorate for doing so. “When I was informed that this year’s Challenge was astronomy themed and help was needed, I was all over it!” she says. Since joining LLNL in 2019, Pruett supports the Astronomy and Astrophysics Analytics Group and Space Science and Security Program, applying data science to topics such as dark matter, dark energy, and space situational awareness. She points out, “At the Lab, we’re using data science and machine learning across so many different fields and for such a diverse range of applications.” With a B.S. in Physics from UC Davis, Pruett currently pursues a Master’s program in Data Analytics at the Air Force Institute of Technology.

Kathleen Schmidt
Applied Statistician
As an applied statistician who enjoys tackling interesting problems, Kathleen Schmidt is never bored. “Nearly every field with a quantitative question can benefit from a statistician, so we get to explore a wide variety of science applications,” she says. Schmidt works primarily on two projects: one with messy physics reaction history data collected from older technology, and another where statistical modeling helps optimize materials strength experiments. Her recent publications include modeling for radiation source localization and material behavior in extreme conditions. During 2019–2021, Schmidt served as technical coordinator for the DSI’s seminars and transitioned the series to a virtual format in 2020. She recently spoke at the 4th Annual Reaction History Workshop and has presented at the Lab’s regional Women in Data Science event. She states, “Each data scientist has an individual area of expertise. In coming together as a community, we all have something to contribute.” Schmidt earned a PhD in Applied Mathematics from North Carolina State University before joining the Lab as a postdoctoral researcher in 2016 and converting to full-time staff in 2018.

Katie Lewis
Associate Division Leader
Katie Lewis started working at LLNL in 1998, just days after earning a B.S. in Mathematics from the University of San Francisco. She spent 17 years on a parallel mesh generation project that she ultimately led, and has held numerous leadership positions in the Lab’s Computing, National Ignition Facility, and Weapons and Complex Integration directorates. Today, Lewis serves as Associate Division Leader for the Computational Physics Section of the Design Physics Division where her duties span recruiting, hiring, professional development, workforce planning, staff assessment, and diversity and inclusion efforts. Lewis also leads the Vidya machine learning project in LLNL’s Weapons Simulation and Computing Program, where she applies artificial intelligence techniques to high-performance computing simulations. “AI/ML is proving to be a gamechanger in approaching challenging problems related to scientific computing. Employing these techniques to solve problems more accurately and more quickly will lead to greater scientific discovery,” she states.

Brian Gallagher
Computer Scientist
Mentoring has always been important to Brian Gallagher, who joined the Lab in 2005 after completing his M.S. in Computer Science at UMass Amherst. “I feel extremely grateful for the opportunities I’ve had in my life and the people who have helped me along the way,” he states. After serving as a DSI Data Science Challenge mentor in 2020, Gallagher directs this year’s program for UC Merced and UC Riverside students. “My main goal for the Challenge is to provide an environment where everyone can grow,” he says. “You can see and feel the changes in people from day to day. That’s my favorite part of the experience.” When he’s not working with students, Gallagher leads the Data Science and Analytics Group at LLNL’s Center for Applied Scientific Computing. He contributes to LLNL projects that leverage machine learning for nuclear threat-reduction applications, optimization of feedstock materials, and design of high-entropy alloys. “Because data science is so broadly applicable, I am constantly exposed to new application areas and new people from a variety of backgrounds,” Gallagher explains.

Kelli Humbird
Design Physicist
To understand the science of nuclear weapons without underground testing, researchers at LLNL’s National Ignition Facility conduct inertial confinement fusion (ICF) experiments and optimize target designs for higher energy yields. Design physicist Kelli Humbird has developed machine learning models that accurately predict energy yield and reveal the surprising potential of ovoid-shaped targets. She explains, “This is a great example of what machine learning can do because it has no biases. It directed us to a design space we would not have typically considered.” She presented her research on transfer learning for ICF applications at LLNL’s 2020 Women in Data Science regional conference and says, “I feel really lucky to be a part of such cool science.” A former Lab intern and Livermore Graduate Scholar, Humbird holds a PhD in Nuclear Engineering and Physics from Texas A&M University and recently received her alma mater’s Nuclear Engineering 2020/2021 Young Former Student Award.

Olivia Miano
Data Scientist
With a B.S. in Mathematics and Computer Science from UC San Diego, Olivia Miano was poised to join LLNL in the spring of 2020 as a software developer. Then she heard about the Data Science Immersion Program and immediately signed up. “I knew next to nothing about data science when I first joined the Lab, so almost everything I know I learned during the program,” she says. Under the mentorship of David Buttler and Juanita Ordoñez, Miano explored word embeddings and active learning for context-based entity classification as well as authorship attribution and verification with social media data. A year later, she works on natural language processing projects—including information extraction and authorship verification—for LLNL’s Global Security Computing Applications Division. For Miano, the challenges of applying data science are also what make it exciting. She states, “You need domain knowledge on top of your data science, computer science, and math knowledge. And I’m always eager to learn and willing to tackle a challenging assignment, especially when the work is meaningful like what we do at the Lab.”

Marisol Gamboa
Computer Scientist
Marisol Gamboa thrives at the intersection of solving challenges in unique ways and mentoring the next generation. Over her 18-year career at LLNL, she has honed expertise in software engineering, web applications, and big data analytics by developing solutions for numerous defense and counterproliferation programs—such as tools that help Department of Defense personnel distill, combine, relate, manipulate, and access massive amounts of data in a timely manner. “The many lessons I’ve learned over the years have positioned me to tackle any challenge knowing that I am able to learn quickly and adjust to any situation in real-time,” she says. Gamboa is the Deputy Division Leader for LLNL’s Global Security Computing Applications Division as well as Computing’s Workforce Team Lead. She formerly co-directed the Data Science Summer Institute and created the annual Data Science Challenge with UC Merced. Active in outreach to young women and underrepresented minorities in STEM—including LLNL’s Women in Data Science regional events—Gamboa holds a B.S. in Computer Science from the University of New Mexico.
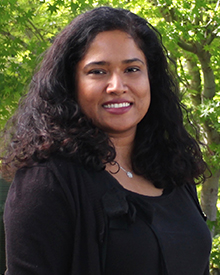
Nisha Mulakken
Biostatistician
Nisha Mulakken’s research lies at the confluence of biology, computer science, and statistics. Her work in LLNL’s Bioinformatics Group includes enhancing the Lawrence Livermore Microbial Detection Array (LLMDA) system with detection capability for all variants of SARS-CoV-2, as well as analyzing mutations in SARS-CoV-2 proteins to support future discovery of therapeutic compounds. In another project, she uses machine learning to trace unethical use of CRISPR technology to the source lab. Mulakken was recently named the new co-director of the Data Science Summer Institute and looks forward to working with the class of 2021. She says, “I hope the students will experience the Lab’s collaborative culture, learn about academic topics and practical applications they may not have been exposed to yet, and genuinely enjoy getting to know each other and their mentors.” A four-time LLNL summer intern and longtime employee, Mulakken holds degrees in genetics and biostatistics.

Harsh Bhatia
Computer Scientist
Harsh Bhatia’s research in scientific visualization is all about seeing the unseen. “In this field, we can develop new techniques that distill extremely complex data into comprehensible visual information,” he states. His wide range of projects include applying topological techniques to understand the behavior of lithium ions, generating topological representations of aerodynamics data, and analyzing and visualizing HPC performance data. Notably, Bhatia and his collaborators won the SC19 Best Paper Award for their work on the Multiscale Machine-Learned Modeling Infrastructure (MuMMI), which predictively models protein interactions that can lead to cancer. He notes, “MuMMI offers a new paradigm that is arbitrarily scalable and promises to solve the problems no other technology can.” Bhatia was a Lawrence Graduate Scholar and an LLNL postdoctoral researcher before joining the Lab’s Center for Applied Scientific Computing full time in 2017. He holds a PhD from the University of Utah’s Scientific Computing and Imaging Institute.

Frank Di Natale
Computer Scientist
Frank Di Natale looks for ways to more easily and effectively harness compute power, especially when a real-world problem is at stake. He says, “Leaning on simulations to better understand our world requires making compute accessible and facilitating sound simulation software and tools.” A notable example is the research described in the SC19 Best Paper. Di Natale and researchers from several organizations developed the novel Multiscale Machine-Learned Modeling Infrastructure (MuMMI) that predictively models the dynamics of RAS protein interactions with lipids in cell membranes. RAS protein mutations are linked to more than 30% of all human cancers. MuMMI’s machine learning algorithm selects lipid “patches” for closer examination while reducing compute resources. As lead author of the winning paper, Di Natale is proud of the team’s accomplishment and excited for MuMMI’s next phase: atomistic-scale protein simulation. “It’s exciting to design a multi-component system that produces the computational techniques that explore science in new ways,” he explains. Di Natale, who came to the Lab in 2016 after a stint at Intel Corporation, has an M.S. in Computer Science from the University of Colorado at Boulder. He is also the PI for the open-source Maestro Workflow Conductor software.

Amar Saini
Data Scientist
Amar Saini lives by the motto “Saving the world, one epoch at a time.” Epoch refers to a learning model making a single pass over its training data. As for saving the world, Saini points to LLNL’s mission that tackles a range of global and national security problems. “In my eyes, using deep learning, machine learning, and artificial intelligence to contribute to these solutions is saving the world,” he says. Saini works mostly with DL and neural networks. Recently he explored a denoising model that removed background noise from voice audio by converting the audio to images, then used neural networks (U-Nets) to remove distortion and convert the image back to audio. His hackathon experiments include voice cloning and altering car color with generative adversarial networks. “Everything in this field is a challenge because we’re constantly researching to find the best methods to train our DL models,” Saini explains. Last fall, he traveled to Copenhagen to give a talk at the ACM Conference on Recommender Systems. Saini is active on the FastAI and PyTorch forums, volunteers with Girls Who Code, and helps organize hackathons. With an M.S. in Electrical Engineering and Computer Science from UC Merced, he joined LLNL in 2019 after a Data Science Summer Institute internship.
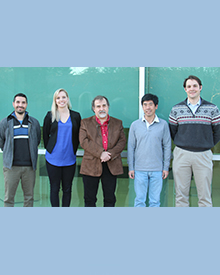
COVID-19 Research Team
Research Scientists
LLNL researchers have identified an initial set of therapeutic antibody sequences, designed in a few weeks using machine learning (ML) and high-performance computing (HPC), aimed at binding and neutralizing SARS-CoV-2, the virus that causes COVID-19. As reported by LLNL News, the research team is performing experimental testing on the chosen antibody designs and working on publishing the results. Pictured here are Dan Faissol (also a member of the DSI Council), Magdalena Franco, Adam Zemla, Edmond Lau, and Tom Desautels. They used two of the Laboratory’s supercomputers—Catalyst and the coincidentally named Corona—and an ML-driven computational platform to design antibody candidates predicted to bind with the SARS-CoV-2 Receptor Binding Domain, narrowing the number of possible designs from a nearly infinite set of candidates to 20 initial sequences predicted to target SARS-CoV-2. “The combination of all of these computational elements, including bioinformatics, simulation, and ML, means that we can flexibly and scalably follow up on mutants with promising predictions as they emerge, effectively using Livermore’s HPC systems,” said project PI Desautels.

Ryan Goldhahn
Staff Scientist
Collaborative autonomous networks have been used in national security, critical infrastructure, and commercial applications. As hardware becomes smaller, faster, and cheaper, new algorithms are needed to collectively make sense of and collaboratively act upon the data collected from sensors. In this context, Ryan Goldhahn says, the sum can be greater than the parts. He explains, “An individual sensor may not be very capable, but the algorithms we develop at LLNL allow large networks of sensors to ‘punch above their weight’ through data fusion and cooperative behaviors.” His team recently filed a patent on a method of decentralized estimation in large autonomous sensor networks using stochastic gradient Markov chain Monte Carlo techniques. Goldhahn, who works in in LLNL’s Computational Engineering Division, was a featured speaker in the DSI’s 2019 seminar series. “The DSI helps keep the Lab on the cutting edge,” he says. “I love exchanging ideas with people who have such amazing technical depth, and solving problems of national significance makes the job that much more fulfilling.” At LLNL since 2015, Goldhahn holds a PhD in Electrical and Computer Engineering from Duke University.

Yong Han
Materials Scientist
Machine learning (ML) and data analytics tools are rapidly proving necessary for materials discovery, optimization, characterization, property prediction, and accelerated deployment. Yong Han is at the forefront of LLNL’s efforts to integrate data science techniques into materials science research and development. For example, he leads a team that uses ML to analyze multimodal data for optimizing feedstock materials. Han explains, “We’re addressing important questions in data sparsity, explainability, reliability, uncertainty, and domain-aware model development.” His recent work in this area includes an njp Computational Materials paper, a Science & Technology Review research highlight, and a DSI research spotlight. “Not all data are created equal. We need to evaluate what data we’re collecting and how we’re collecting them,” Han states. He emphasizes that domain scientists and data scientists will benefit from working closely together, adding, “I envision permeation of data science tools in all of our projects at the Lab.” Han holds a PhD in Chemistry from UC Santa Barbara and joined LLNL in 2005.

DSSI class of 2019
Aspiring Data Scientists
The DSSI class of 2019—31 students in all—were selected from a highly competitive applicant pool of more than 1,400. While at LLNL, they had access to LLNL’s HPC resources, participated in Grand Challenge team exercises, and displayed their research posters at LLNL's student poster symposium. These bright students are among the next generation of promising data scientists, and we look forward to seeing their careers develop.
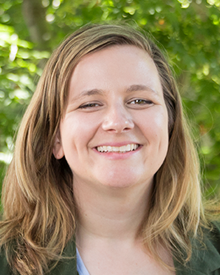
Cindy Gonzales
Data Scientist
Cindy Gonzales joined LLNL intending to continue her career as an administrator with the Computing Scholar Program, working with summer students during their internships. Then she attended a machine learning (ML) seminar, and the rest is history. She says, “I was taking an introductory statistics course as a part-time student when I learned what ML was. I thought, I could do this.” For two and a half years, Gonzales juggled a demanding workload: interning with data scientists, learning from mentors, supporting the DSI, coordinating the Scholar Program, and attending school part time. She earned her B.S. in Statistics from Cal State East Bay before beginning a distance-learning M.S. in Data Science at Johns Hopkins. Today, Gonzales uses ML to detect objects in satellite imagery—work she will present at the Applied Imagery and Pattern Recognition workshop in October. She explains, “Data science is such a diverse field, which makes it both exciting and challenging. You need a background in many different areas such as computer science plus domain knowledge. These skills will open doors to other scientific domains.”

Amanda Minnich
Machine Learning Research Scientist
Dr. Amanda Minnich’s passion for socially meaningful and scientifically interesting projects converges with her machine learning (ML) and data mining expertise in the Accelerating Therapeutics for Opportunities in Medicine (ATOM) Consortium. Co-founded by LLNL, ATOM aims to accelerate drug development and discovery. “We want to show that ML has a place in the pharmaceutical world,” says Minnich. “I use historical drug data from pharmaceutical companies to build ML models that predict key pharmacokinetic and safety parameters.” Minnich also applies her skills to community outreach: She has served as a Girls Who Code mentor, organized a speed-mentoring session at LLNL’s 2019 Women in Data Science regional event, and recently spoke at the DSI-co-sponsored women’s lunch at the 2019 Conference on Knowledge Discovery and Data Mining. Minnich joined LLNL’s Global Security Computing Applications Division after meeting recruiters at the Grace Hopper Celebration (GHC), where she was a GHC14 scholar and now co-chairs the Artificial Intelligence Track. She holds a BA in integrative biology from UC Berkeley and an MS and PhD in computer science from the University of New Mexico.

Brian Giera
Materials Engineer
Brian Giera thrives on problems that blend innovation and multidisciplinary teamwork, like his latest project that optimizes production of carbon capture technology with machine learning (ML). His team has created microcapsules only a few hundred micrometers in diameter—“They look like blue caviar,” Giera says—that absorb CO2 from the atmosphere. Recently featured in Lab on a Chip, the project uses ML to unlock real-time monitoring and sorting of the microcapsules, reducing production time and expenses while increasing the quality of the collected product. Giera states, “It was fun to have a computation-centric project and produce something tangible in the lab. We worked with experimentalists on an actual microfluidic device.” The system is portable to other microencapsulation devices and microfluidic production systems that can benefit from automation. With a background in the food and fragrance manufacturing industry, Giera holds a PhD in chemical engineering from UC Santa Barbara. He is active in LLNL’s Abilities Champions employee resource group and regularly mentors interns, noting, “Students are an excellent source of collaboration.”
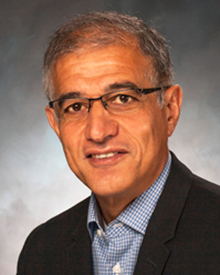
Ghaleb Abdulla
Computer Scientist
Since joining LLNL in 2000, Ghaleb Abdulla has embraced projects that depend on teamwork and data sharing. His tenure includes establishing partnerships with universities seeking LLNL’s expertise in HPC and large-scale data analysis. He supported approximate queries over large-scale simulation datasets for the AQSim project and helped design a multi-petabyte database for the Large Synoptic Survey Telescope. Abdulla used machine learning (ML) to inspect and predict optics damage at the National Ignition Facility, and leveraged data management and analytics to enhance HPC energy efficiency. Recently, he led a Cancer Registry of Norway project developing personalized prevention and treatment strategies through pattern recognition, ML, and time-series statistical analysis of cervical cancer screening data. Today, Abdulla is co-PI of the Earth System Grid Federation—an international collaboration that manages a global climate database for 25,000 users on 6 continents. “The ability to move between different science domains and work on diverse data science challenges makes LLNL a great place to pursue a career in data science,” he says. Abdulla holds a PhD in computer science from Virginia Tech.

CASC ML team
Computer Scientists
As machine learning (ML) research heats up at LLNL, a team of computer scientists from the Center for Applied Scientific Computing (CASC) is leading the way. Pictured here are Harsh Bhatia, Shusen Liu, Bhavya Kailkhura, Peer-Timo Bremer (also a member of the DSI Council), Jayaraman Thiagarajan, Rushil Anirudh, and Hyojin Kim. Their research was recently featured in LLNL’s magazine, Science & Technology Review. As the cover story, “Machine Learning on a Mission,” explains, ML has important implications for scientific data analysis and for the Lab’s national security missions. This CASC team takes a bidirectional approach to ML, both advancing underlying theory and solving real-world problems—an effort that includes scaling algorithms for supercomputers and developing ways to analyze different types and varying volumes of data. Bremer states, “Commercial companies don’t solve scientific problems, just as national labs don’t optimize selections of movie reviews. So we build on commercial tools to create the techniques we need to analyze data from experiments, simulations, and other sources.”

Laura Kegelmeyer
Scientist/Engineer
Laura Kegelmeyer embraces her role as a problem solver. Since arriving at LLNL in 1988, she has brought her expertise to bear on image processing and analysis—first in biomedical applications, such as DNA mapping and breast cancer detection, and now at the National Ignition Facility (NIF), home of the world’s most energetic laser. Her Optics Inspection team combines large-scale database integration with custom machine learning algorithms and other data science techniques to analyze images captured throughout NIF’s 192 beamlines. This inspection process informs an automated “recycle loop” that extends optic lifetimes. Based on this work and previous involvement with Women in Data Science (WiDS) events, Kegelmeyer was invited to speak at the 2019 WiDS conference. “It’s an amazing opportunity to present an example of applying machine learning to ‘big science.’ NIF’s exploration of physical phenomena under extreme conditions has far-reaching impact across the globe and for future generations,” she says. “I hope to inspire data scientists to use their skills to address challenges in exciting scientific areas.” Kegelmeyer holds degrees in Biomedical Engineering and Electrical Engineering from Boston University.
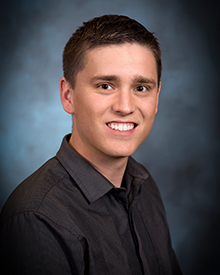
Brenden Petersen
Research Scientist
Brenden Petersen isn’t content merely applying advanced data science methods to real-world problems. He’d rather tackle challenges where, he says, “the state-of-the-art doesn’t cut it.” Since joining LLNL’s Computational Engineering Division in 2016, he pursues deep reinforcement learning (RL) solutions for many fields including cybersecurity, energy, and healthcare (see DSI workshop slides [PDF]). Whereas deep learning traditionally addresses prediction problems, RL solves control problems. He explains, “RL provides a framework for learning how to behave in a task-completion scenario. Working in the field feels very goal-oriented, even competitive. Each application is a new personal challenge.” Petersen recently launched an RL reading group to help other LLNL staff get started in the field. “At the first meeting, I recognized only about 20% of the attendees, which was awesome! A major goal of the group, and DSI as a whole, is to connect researchers across the Lab,” he states. Petersen earned his biomedical engineering PhD through a joint program at UC Berkeley and UC San Francisco.
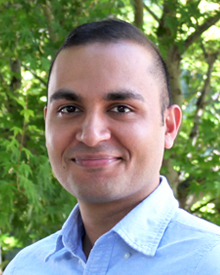
Bhavya Kailkhura
Computer Scientist
Kailkhura thrives on solving challenging problems in data science, focusing on improving the reliability and the safety of machine learning systems. “Reliability and safety in AI should not be an option but a design principle,” he states. “The better we can address these challenges, the more successful we will be in developing useful, relevant, and important ML systems.” Kailkhura also pursues mathematical solutions to open optimization problems, including a novel sphere-packing theory. He is building provably safe, explainable deep neural networks to enable reliable learning in applications for materials science, autonomous drones, and inertial confinement fusion. Thanks to his efforts with gradient-free algorithms and experiment designs, LLNL is the only national lab with research accepted at two high-profile venues—NIPS and JMLR—in 2018. Prior to joining LLNL’s Center for Applied Scientific Computing, Kailkhura attended Syracuse University where his PhD dissertation won an all-university prize. Recently, he co-authored the book Secure Networked Inference with Unreliable Data Sources.

Kassie Fronczyk
Applied Statistics Group Leader
Fronczyk is a “total nerd” whose multifaceted job makes her an ideal panelist for the Women in Statistics and Data Science conference, where she recently discussed research opportunities at national labs. Fronczyk leads LLNL’s Applied Statistics Group while providing statistical analysis and uncertainty quantification for several projects, including a warhead life-extension program and the U.S. Nuclear Detection System. “I love learning new things and tackling interesting problems,” states Fronczyk. “Standard approaches rarely work on real-world data, so finding the right tool for the job often means exploring new methods and combining or modifying others.” She brings this creative mentality to on- and offsite collaborations, such as with the Innovations and Partnerships Office and the Institute of Makers of Explosives Science Panel. She also sits on LLNL’s Engineering Science & Technology Council, manages two seminar series (including DSI’s), and co-organized DSI’s inaugural workshop. Fronczyk holds a PhD in statistics and stochastic modeling from UC Santa Cruz.

Jose Cadena Pico
Postdoctoral Researcher
Cadena Pico enjoys the discovery process when analyzing new data sets, despite the difficulties in preparing data before building machine learning models. “Often a data set is incomplete or contains errors from different sources. Sometimes its size makes it difficult to extract knowledge,” he says. “Solving these challenges and knowing that I’m helping other researchers advance their work is very gratifying.” Once a PhD student at Virginia Tech, Cadena Pico now contributes to LLNL’s brain-on-a-chip project by studying complex networks among brain cells. He also investigates ways to detect anomalous activity in networks, and his recent work—developing a method for finding clusters of under-vaccinated populations to inform public health resources—was presented at the 24th KDD Conference. Formerly a three-time LLNL summer intern, Cadena Pico values ongoing education: “I like to keep learning about different research domains while developing a data science skill set applicable to many problems of global importance.”

DSSI class of 2018
Aspiring Data Scientists
The DSSI class of 2018—26 students in all—were selected from a highly competitive applicant pool of more than a thousand. While at LLNL, they participated in Grand Challenge team exercises and displayed their research posters at the DSI’s summer workshop. These bright students are among the next generation of promising data scientists, and we look forward to seeing their careers develop.

Rushil Anirudh
Research Scientist
With a PhD in computer vision and machine learning, Anirudh joined LLNL’s Center for Applied Scientific Computing in 2016. He enjoys the challenges of an exponentially growing field, noting, “Something on a whiteboard today is likely to end up being used by someone within a few months.” Anirudh develops convolutional neural networks that can complete computed tomography (CT) images when the scanned object is only partially visible. His team’s paper, “Lose the Views: Limited Angle CT Reconstruction via Implicit Sinogram Completion,” is one of only 7% selected for a spotlight presentation at the 2018 Computer Vision and Pattern Recognition conference. Anirudh’s related work with generative adversarial networks was recently featured in NVIDIA’s developer blog. “I am very glad the Lab has the DSI,” says Anirudh. “A central institute that brings together everyone working on similar ideas is a great step toward becoming a leader in artificial intelligence and machine learning.”

T. Nathan Mundhenk
Computer Scientist
Mundhenk enjoys “nerding around” in LLNL’s Computational Engineering Division, especially when it comes to research aimed at improving people’s lives. With a PhD in computer science from the University of Southern California, he works on projects that use LLNL’s powerful computing capabilities to advance neural network technologies. Mundhenk recently co-authored a paper, “Improvements to Context Based Self-Supervised Learning,” which was accepted to the 2018 Computer Vision and Pattern Recognition conference. His team is developing a state-of-the-art technique for refining unsupervised deep learning. In their method of self-supervision, a deep neural network can be pre-trained on a large generic dataset before training on a small labeled dataset, resulting in better accuracy (e.g., of image recognition) in the latter. “The entire field of artificial intelligence is bursting with new innovation,” says Mundhenk. “It’s challenging to keep up with the extraordinary pace of research, but also very exciting to be part of it.”

Marisa Torres
Senior Bioinformatics Software Developer
Since joining LLNL in 2002, Torres has combined her love of biology with coding. She serves as lead bioinformatics software developer on biosecurity projects supporting the Global Security Program. Her team is building the Gene Surprise Toolkit, which determines biothreat severity and detects potential genetic engineering of pathogens. In addition, Torres contributes to the Accelerating Therapeutics for Opportunities in Medicine consortium. The project aims to accelerate the drug discovery pipeline by building predictive, data-driven pharmaceutical models. In March 2018, Torres organized a regional symposium in conjunction with Stanford University’s Women in Data Science conference. She also encourages local middle school students to explore computer science through the Girls Who Code program and mentors student interns for LLNL’s Data Science Summer Institute (DSSI). “I’m interested in collaborating across domains with similar data analysis needs,” says Torres. “I look forward to strengthening networking and educational opportunities through DSI, especially for the DSSI.”