
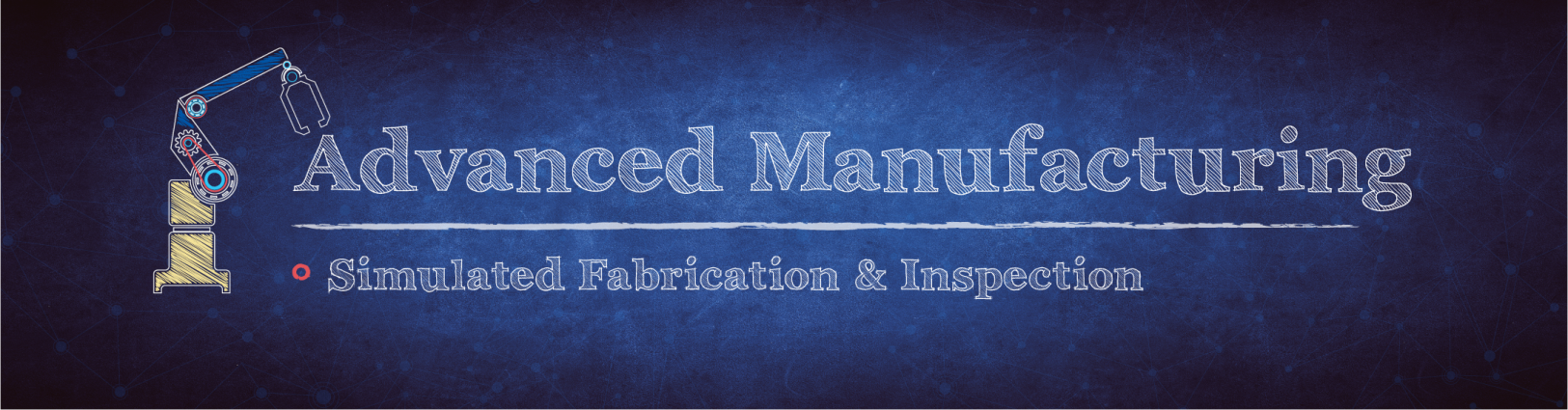

“A digital twin that captures real artifacts and manufacturing variability can be used to virtually build, simulate, and evaluate potential parts.”
– Brian Giera
In service to LLNL’s national security mission, scientists and engineers are constantly seeking new ways to improve manufactured parts and materials by optimizing production, predicting performance, and ensuring reliability. In particular, inspection is a significant bottleneck in NNSA’s manufacturing pipeline and can exceed fabrication costs many times over.
A new capability has emerged from a Laboratory Directed Research and Development project focusing on improving manufacturing process performance and component quality: Digital twins (DTs) are virtual representations of physical systems, combining physics-based and data-driven models. DTs can simulate the advanced manufacturing pipeline, speeding up development time and reducing the costs and risks associated with using real equipment and materials. Research engineer Brian Giera states, “A digital twin that captures real artifacts and manufacturing variability can be used to virtually build, simulate, and evaluate potential parts.”
According to Giera, DTs add a new dimension to both process- and part-level fabrication and inspection. He explains, “Complete manufacturing and inspection require people, time, effort, and equipment. Incredibly intricate parts may have features beyond what traditional inspection techniques can detect. Looking at the whole process beyond the part itself, input instructions and other factors may affect part performance. Integrating a digital twin framework into the manufacturing pipeline will help us optimize both fabrication and inspection.” For example, a DT of a manufacturing process will use the same inputs as its physical counterpart, simulate machine motions and states, and output a DT of each part made.
As Giera’s team envisions, process-level DTs will replicate real manufacturing systems from command inputs all the way through data stream outputs. These models will capture an operation digitally with empirical data, span the necessary length- and time-scales for the duration of the build, and make data-driven refinements in real time. In addition to providing insights on individual parts, process-level DTs can inform machine health and behavior, troubleshoot complex toolpaths, identify equipment flaws, and train operators virtually.
Part-level DTs can replicate the physical part before it is made, enabling virtual evaluation of geometric tolerancing, stress-versus-strain measurements, and other performance and quality tests. Creating a digital representation opens the door to better predictions, and parts can be produced with fewer defects. The entire manufacturing pipeline could scale more efficiently and effectively.
Giera’s team plans to incorporate machine learning (ML) and virtual reality (VR) into this pipeline. “Machine learning can capture the salient features, including errors, of the manufacturing process,” he explains. “We can inject those features into the digital twin to add variability, then extract trends from datasets that grow over time, which will help us make more realistic models.” Ultimately, combining physics expertise with ML techniques will produce scientifically driven DT models that leverage both process- and part-level data. In a VR environment, operators can perform inspections with complex datasets and collaborate without being co-located.
The team is developing DTs for cushion-like parts made via direct ink writing systems acquired from industry partner Aerotech. Giera points out that DTs can benefit any manufacturing technique including laser-driven powder bed fusion and other forms of 3D printing. He says, “The digital twin concept and framework are bigger than any single manufacturing method. We envision broader use among the Department of Energy community, industry, and academia.”
Team Acknowledgments
In addition to Giera, LLNL researchers include Ziad Ammar, Brian Au, Peer-Timo Bremer, Nicholas Calta, Alex Caviness, Kyle Devlugt, Terence Hall, Haichao Miao, Michael Johnson, Dylan Kline, Seth Watts, Todd Weisgraber, Brian Weston, and Michael Zelinski.