Aug. 23, 2023

Data Science Challenge Tackles ML-Assisted Heart Modeling
For the first time, students from the University of California’s Merced and Riverside campuses joined forces for the two-week Data Science Challenge at LLNL, tackling a real-world problem in machine learning (ML)-assisted heart modeling. Held during July 10–21, the event brought together 35 UC students—ranging from undergraduates to graduate-level students from a diversity of majors—to work in groups to solve four key tasks, using actual electrocardiogram data to predict heart health. According to organizers, the purpose of the challenge was to give students a taste of the broad scope of work that goes on at a national laboratory and provide them with experience working in interdisciplinary teams.
“One of my main goals is developing the students’ technical skills, but I also want to get people excited about going to grad school who hadn’t thought about it yet,” said Brian Gallagher, who co-directed the DSC program alongside Cindy Gonzales. “I want to get people thinking about a science career, not just a web development career or software engineering career. The students have been super engaged and interested in career paths and job progressions. Many have asked how to get an internship, which I take as a good sign.” UC Merced also covered the event on their website.

Register Now for DOE Data Days
The 2023 DOE Data Days (D3) Workshop returns to LLNL in person on October 24–26. Registration is open through October 16. Contact felker7 [at] llnl.gov (Lisa Felker) for an invitation, and visit the D3 website for additional details.
D3 provides a forum to collaborate on data management ideas and strategies and build an actionable plan forward to drive innovation and progress across DOE and NNSA. The workshop brings together data management practitioners, researchers, and project managers from DOE and the national labs to promote data management as a means to higher quality and more efficient research and analysis. Presentations and posters from the DOE data management community will cover five themes:
- Data Intensive Computing
- Cloud and Hybrid Data Management
- Data Access, Sharing, and Sensitivity
- Data Curation and Metadata Standards
- Data Governance and Policy
Breakout sessions will provide the opportunity to delve further into high interest topics in DOE data management and facilitate collaboration across institutions. Keynote speakers from DOE and other government agencies will highlight policy and implementation strategy success in their work.
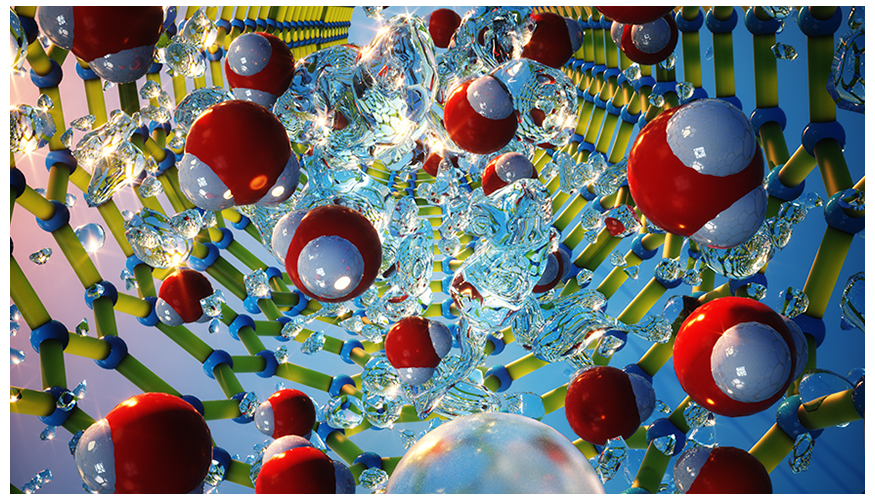
ML Reveals Refreshing Understanding of Confined Water
LLNL scientists combined large-scale molecular dynamics simulations with ML interatomic potentials derived from first-principles calculations to examine the hydrogen bonding of water confined in carbon nanotubes (CNTs). They found that the narrower the diameter of the CNT, the more the water structure is affected in a highly complex and nonlinear fashion. The research appears on the cover of The Journal of Physical Chemistry Letters. The hydrogen-bond network of confined water in nanopores deviates from the bulk liquid, yet looking into the changes is a significant challenge. In the recent study, the team computed and compared the infrared spectrum of confined water with existing experiments to reveal confinement effects.
“Our work offers a general platform for simulating water in CNTs with quantum accuracy on time and length scales beyond the reach of conventional first-principles approaches,” said LLNL scientist Marcos Calegari Andrade, lead author of the paper. The team developed and applied a neural network interatomic potential to understand the hydrogen bonding of water confined in single-walled CNTs. This potential allowed an efficient examination of confined water for a wide range of CNT diameters at time and length scales beyond-reach of conventional first-principles approaches while retaining their computational accuracy.

Explainable AI Can Enhance Scientific Workflows
As ML and AI tools become more widespread, a team of researchers in LLNL’s Computing and Physical and Life Sciences directorates are trying to provide a reasonable starting place for scientists who want to apply ML/AI, but don’t have the appropriate background. The team’s work grew out of a Laboratory Directed Research and Development project on feedstock materials optimization, which led to a pair of papers (linked below) about the types of questions a materials scientist may encounter when using ML tools, and how these tools behave. Explainable artificial intelligence (XAI) is an emerging field that helps interpret complicated ML models, providing an entry point to new applications. XAI may use tools like visualizations that identify which features a neural network finds important in a dataset, or surrogate models to explain more complex concepts.
- A strategic approach to machine learning for material science: how to tackle real-world challenges and avoid pitfalls in Chemistry of Materials – Piyush Karande, Brian Gallagher, and T. Yong-Jin Han
- Explainable machine learning in materials science in npj Computational Materials – Xiaoting Zhong, Gallagher, Shusen Liu, Bhavya Kailkhura, Anna Hiszpanski, and Han

Recent Research
Preprints, full text, PDFs, or conference abstracts are linked where available.
29th ACM SIGKDD Conference on Knowledge Discovery and Data Mining: Graph learning in physical-informed mesh-reduced space for real-world dynamic systems – Yeping Hu, Bo Lei, Victor M. Castillo
IEEE Transactions on Computers:
- ARETE: accurate error assessment via machine learning-guided dynamic-timing analysis – Giorgis Georgakoudis with colleagues from Queen's University Belfast and U-blox
- Improving diversity with adversarially learned transformations for domain generalization – Rushil Anirudh, Jayaraman Thiagarajan, Bhavya Kailkhura with colleagues from Arizona State University
Joint Statistical Meetings (JSM2023): Enforcing hierarchical label structure for multiple object detection in remote sensing imagery – Laura Wendelberger, Cindy Gonzales, Wesam Sakla
Journal of Chemical Theory and Computation: Machine learning-driven multiscale modeling: bridging the scales with a next-generation simulation infrastructure – Helgi Ingólfsson, Tomas Oppelstrup, Timothy Carpenter, Sergio Wong, Xiaohua Zhang, Joseph Chavez, Brian Van Essen, Peer-Timo Bremer, James Glosli, Felice Lightstone, Frederick Streitz, with colleagues from Los Alamos National Laboratory and San Jose State University (Image at left: Lipid densities and coarse-grained placements from a continuum simulation.)
Journal of Computational Physics: Accelerating discrete dislocation dynamics simulations with graph neural networks – Nicolas Bertin, Fei Zhou
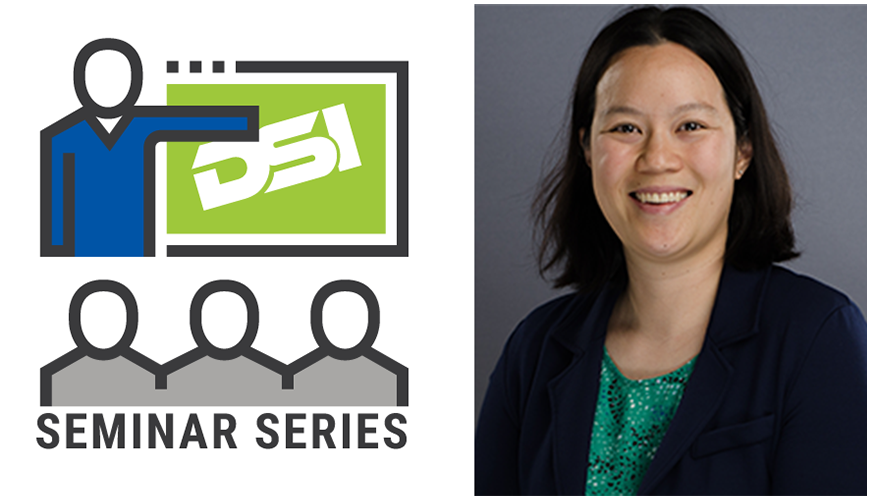
Seminar Explores Tensor Factorization in Biomedical Scenarios
At the DSI’s July 28 seminar, Dr. Joyce Ho from Emory University presented “Tensor Factorization for Biomedical Representation Learning.” She explained how tensors can succinctly capture patient representations from electronic health records to deal with missing and time-varying measurements while providing better predictive power than deep learning models. Ho also discussed how tensor factorization can be used for learning node embeddings for both dynamic and heterogeneous graphs, and illustrated their use for automating systematic reviews.
Ho is an Associate Professor in the Computer Science Department at Emory University. She received her PhD in Electrical and Computer Engineering from the University of Texas at Austin. Her research focuses on the development of novel machine learning algorithms to address problems in healthcare such as identifying patient subgroups or phenotypes, integration of new streams of data, fusing different modalities of data, and dealing with conflicting expert annotations.
The next seminar will be held on September 7. Dr. Doug Bowman from Virginia Tech will present “Quantifying the Benefits of Immersion in Virtual Reality.” Speakers’ biographies and abstracts are available on the seminar series web page, and many recordings are posted to the YouTube playlist. To become or recommend a speaker for a future seminar, or to request a WebEx link for an upcoming seminar if you’re outside LLNL, contact datascience [at] llnl.gov (datascience[at]llnl[dot]gov).
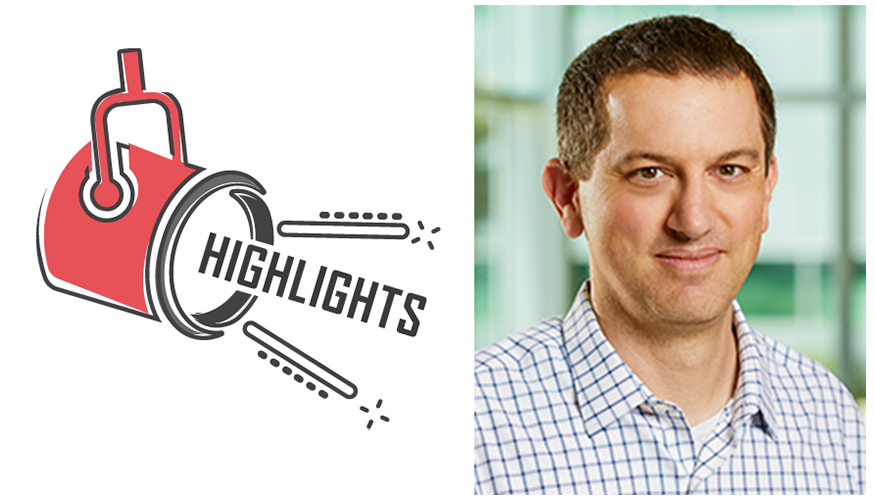
Meet an LLNL Data Scientist
Andrew Gillette’s mathematics career has evolved from pure theory to applied math, and now it also includes machine learning, cognitive simulation, and other data science techniques. The intersection of these fields poses interesting questions and unique possibilities. He states, “Large numerical datasets appear all over the Lab and in all kinds of sciences, so one challenge is figuring out the right approach for modeling the data. How much data do you need, and is the data you already collected enough?” With projects ranging from implicit neural representations in 3D visualization to artificial intelligence in space science, Gillette says a mathematical perspective is necessary in data-driven problems and among LLNL’s multidisciplinary teams. This summer he is mentoring a student who is using reinforcement learning to guide adaptive mesh refinement. “Whether it’s through publications, the code I write, or people I mentor, my work at the Lab will have an impact,” he notes. Gillette holds a PhD in Mathematics from the University of Texas at Austin. He was a tenured professor at the University of Arizona before joining LLNL in 2019.