June 6, 2023

The DSI Turns Five!
Since the DSI’s founding in 2018, the Lab has seen tremendous growth in its data science community and has invested heavily in related research. Five years later, the DSI has found its stride with a multipronged strategy of raising awareness about the field, encouraging partnerships across the community, supporting researchers, and nurturing the next generation of data scientists. A new article chronicles the DSI’s successes and influence both at the Lab and in the external data science community.
Michael Goldman, who directed the DSI until this April, says, “The accomplishments of DSI are not mine. This is a collective effort led by many individuals who have the passion to see data science thrive at LLNL.” DSI Council member Ana Kupresanin adds, “The DSI is uniquely experimental in that it spans all parts of the Lab. It provides a sense of belonging to people who do similar kinds of work. Outside of LLNL, our external engagements are a platform that tells the world who we are and what we work on.” To celebrate this milestone, check out the photo album and redesigned home page.

Video: Data Science Meets Fusion
LLNL’s historic fusion ignition achievement on December 5, 2022, was the first experiment to ever achieve net energy gain from nuclear fusion. However, the experiment’s result was not actually that surprising. A team leveraging data science techniques developed and used a landmark system for teaching artificial intelligence (AI) to incorporate and better account for different variables and experimental scenarios, creating specific parameters for simulations leading up to the successful experiment.
In a new video, LLNL researchers Jayaraman Thiagarajan, Kelli Humbird, and Luc Peterson explain how machine learning (ML) and AI models help advance scientific discovery, how the process of cognitive simulation (CogSim) predicted the successful fusion ignition shot, and the future of this predictive technology. The video includes a softball animation (image at left) that acts as a metaphor for the comparative sophistication of AI, ML, and CogSim.

New SambaNova AI Hardware to Support CogSim Research
LLNL and SambaNova Systems have announced the addition of a spatial data flow accelerator into the Livermore Computing Center, part of an effort to upgrade the Lab’s CogSim program. LLNL will integrate the new hardware to further investigate CogSim approaches combining AI with high-performance computing—and how deep neural network hardware architectures can accelerate traditional physics-based simulations as part of the National Nuclear Security Administration’s Advanced Simulation and Computing program. The Lab is expected to use the SambaNova AI systems to improve the fidelity of models and manage the growing volumes of data to improve overall speed, performance and productivity for stockpile stewardship applications, fusion energy research and other basic science work.
"Multiphysics simulation is complex,” says LLNL Informatics Group Leader Brian Van Essen (pictured with LLNL Chief Technical Officer Bronis de Supinski). “Our inertial confinement fusion experiments generate huge volumes of data. Yet, connecting the underlying physics to the experimental data is an extremely difficult scientific challenge. AI techniques hold the key to teaching existing models to better mirror experimental models and to create an improved feedback loop between the experiments and models. The SambaNova system will help us create these cognitive simulations.”
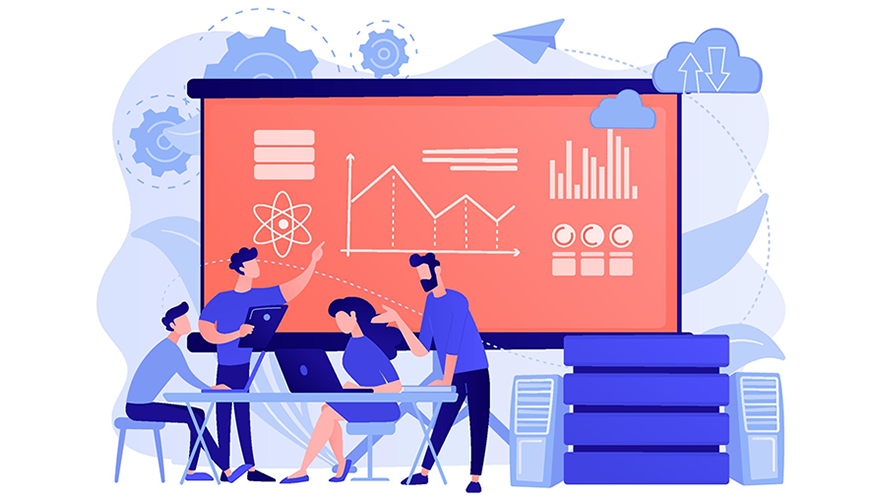
Consulting Service Infuses Lab Projects with Data Science Expertise
A key advantage of LLNL’s culture of multidisciplinary teamwork is that domain scientists don’t need to be experts in everything. Physicists, chemists, biologists, materials engineers, climate scientists, computer scientists, and other researchers regularly work alongside specialists in other fields to tackle challenging problems. The rise of Big Data across the Lab has led to a demand for data science knowledge at any or all stages of a team’s project.
Led by applied statisticians Jason Bernstein and Kathleen Schmidt, the Data Science Institute’s Consulting Service (DSICS) offers statistical and machine learning expertise to Lab research teams on a short-term basis. These consultations can turn into long-term collaborations, such as Laboratory Directed Research and Development projects, and help strengthen ties across the Lab. Bernstein explains, “Consultants can help determine how many experiments need to be completed, or suggest methods to analyze already collected data. Additionally, the DSICS provides a way for data scientists to meet new people at LLNL, learn about the mission areas they work in, and potentially work in those areas themselves. The consulting service often helps the consultant as much as the consultee.”

CASIS Workshop Registration and Call for Abstracts
LLNL’s Center for Advanced Signal and Image Sciences is hosting the CASIS 2023 Workshop on August 2–3 to explore the latest advancements in signal and image sciences. The free event is open to engineers, scientists, and students interested in signal and image sciences, and it will be held at the Livermore Valley Open Campus (LVOC). Attendees can enjoy outstanding presentations, connect with experts across a broad range of topics, and engage in stimulating discussions. The agenda includes talks, interactive poster sessions, and complimentary coffee and lunch breaks. Invited speakers will discuss fusion ignition, large language models, and quantum computing. Abstracts for talks and posters are due June 25. Register for the workshop by July 25. Visit the CASIS website for the agenda, workshop tracks, organizing committee, directions to LVOC, and more.

Patent Applies ML to Industrial Control Systems
An industrial control system (ICS) is an automated network of devices that make up a complex industrial process. For example, a large-scale electrical grid may contain thousands of instruments, sensors, and controls that transfer and distribute power, along with computing systems that capture data transmitted across these devices. Monitoring the ICS network for new device connections, device performance, or adversarial attacks requires sophisticated data analysis. LLNL researchers Brian Kelley, Indrasis Chakraborty, Brian Gallagher, and Dan Merl recently published a patent (pending) for a novel ML framework that discovers and predicts key data about networked devices. Keeping a data-driven eye on an ICS helps ensure its reliability and security.
Kelley explains, “By training the ML model on a variety of datasets across different ICS types, such as data from large utility companies, we want it to learn about the characteristics of those systems. Then when our model is presented with data from a system it hasn’t seen before, it could recognize relevant devices and tell us about the devices’ provenance or metadata.” The pending patent enables the technology to be easily adopted in many use cases: public utilities, building systems, sensors that send and receive signal data, and more.

Notable Top 25% Paper at ICLR 2023
LLNL ML researcher Jayaraman Thiagarajan and two University of Michigan collaborators were recognized at the International Conference on Learning Representations (ICLR) for a notable top 25% paper. The paper, “A Closer Look at Model Adaptation using Feature Distortion and Simplicity Bias,” focuses on designing adaptation protocols from pretrained representations in order to improve ML model generalization and safety. Linear probing and fine-tuning protocols can be effectively combined to improve transfer learning. This paper proposes to view model adaptation from the dual perspective of feature distortion and simplicity bias and designs new transfer learning protocols that lead to models that are not only accurate but also safe (e.g., handling corruptions, out-of-distribution generalization, anomaly rejection, adversarial robustness). The team was able to verify the effectiveness of the proposed protocols with large-scale representation learners (e.g., CLIP and SimCLR) and several benchmark datasets. (Image at left: Accurate and safe adaptation of pre-trained representations is a central component of modern AI systems.)
“With the advent of internet-scale foundation models, it has become imperative to develop tools that can effectively utilize those models in AI system design. It is indeed an honor that this timely work has been featured as a spotlight at this prestigious conference, and this definitely encourages us to further pursue this exciting direction of research,” says Thiagarajan.
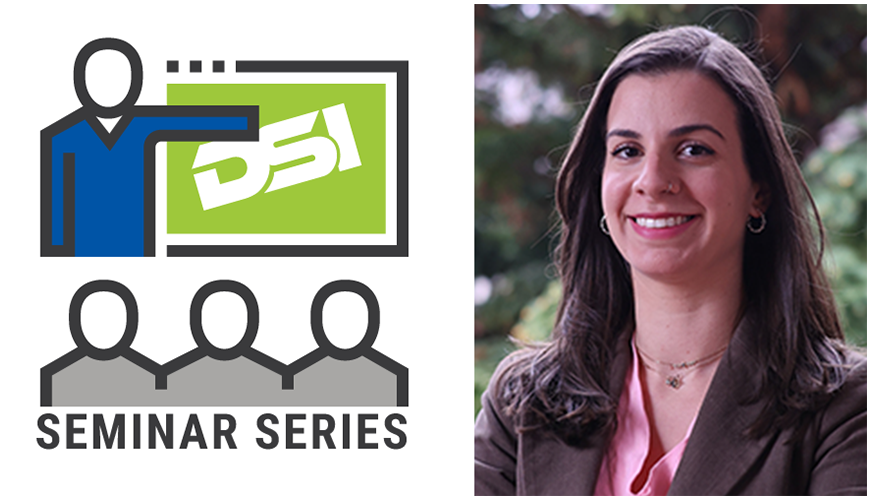
Virtual Seminar Focuses on PDEs and UQ
At the DSI’s May 18 technical seminar, Dr. Katiana Kontolati from Johns Hopkins University presented “Leveraging Latent Representations for Predictive Physics-Based Modeling and Uncertainty Quantification (UQ).” She showcased approaches that leverage latent representations of high-dimensional data to improve the performance of surrogate models and enable UQ for complex partial differential equation (PDE) applications. The talk focused on inverse problems and the development of a manifold-based approach for the probabilistic parameterization of nonlinear PDEs; the Latent Deep Operator Network for training neural operators on latent spaces; and transfer learning for conditional shift in PDE regression.
Kontolati received her PhD from the Department of Civil and Systems Engineering at Johns Hopkins University in 2023. Her doctoral research revolves around physics-informed ML with a focus on high-dimensional surrogate modeling and UQ in physics-based and engineering problems involving nonlinear PDEs under uncertainty.
Speakers’ biographies and abstracts are available on the seminar series web page, and many recordings are posted to the YouTube playlist. To become or recommend a speaker for a future seminar, or to request a WebEx link for an upcoming seminar if you’re outside LLNL, contact datascience [at] llnl.gov (datascience[at]llnl[dot]gov).
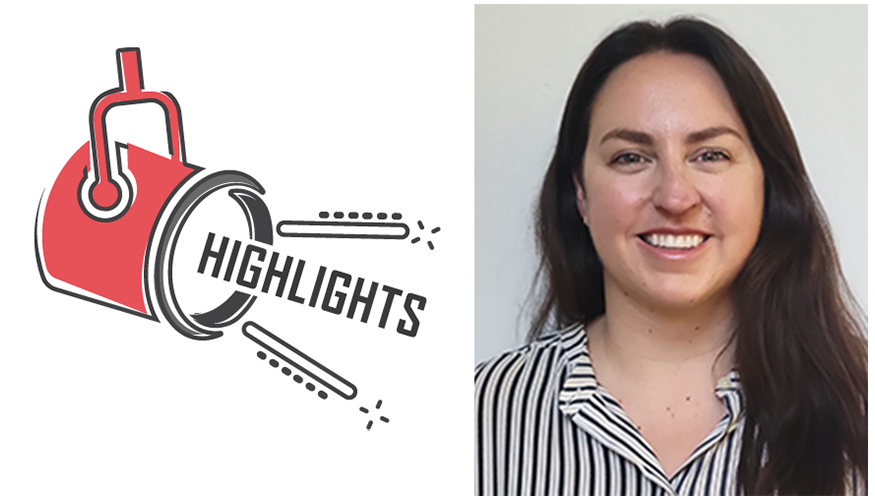
Meet an LLNL Data Scientist
A crucial aspect of data science—particularly at LLNL and across the Department of Energy (DOE)—is the management of big data across different domain expertise and programs. Since joining the Lab’s geophysical monitoring programs in 2019, Rebecca Rodd has focused on data cleaning and ingestion, developing geophysical data management standards, and building data infrastructure for several of NNSA’s Defense Nuclear Nonproliferation R&D projects. “Over the last decade, innovation in data management techniques and tools has led to improvements in data storage, rapid data transfer, integrated data management systems, cloud and hybrid computing, and other areas,” she explains. “The DOE has many data management successes, and applying them to geophysical programs and datasets is exciting and challenging due to the multi-laboratory and multi-phenomenology requirements, solving multi-lab access control and varied security policies, and inconsistent metadata standards across and within domain areas.” Rodd thrives on learning new technologies and in 2022 assumed leadership of the annual DOE Data Days workshop (D3), which brings together data management practitioners, researchers, and project managers to promote data management for higher quality, more efficient R&D across the DOE complex. She notes, “Data management often does not get as much attention at data-focused DOE meetings, so D3 offers a place for more collaboration in this area.” Rodd holds a B.S. in Geology from UC Davis and an M.S. in Geosciences from the University of North Carolina at Chapel Hill.