Feb. 24, 2023

Register for Hybrid WiDS Livermore on March 8
The annual Women in Data Science (WiDS) conference returns on Wednesday, March 8. LLNL will again host a regional event in conjunction with the worldwide conference. The all-day WiDS Livermore event is free and will be presented in a hybrid format at the Livermore Valley Open Campus (LVOC) and via WebEx. Everyone is welcome to attend. Register by February 27.
Along with plenty of food and networking opportunities, WiDS Livermore will include a livestream of the Stanford conference where LLNL WiDS Ambassador Marisa Torres has been invited to speak to the global audience. Returning this year is the popular “speed mentoring” session, where mentees rotate through breakout rooms to speak with mentors. Attendees can sign up to be mentors or mentees during registration.
To mark LLNL’s sixth year of WiDS participation, the Livermore event will include a fireside chat between Dona Crawford, board chair of the Livermore Lab Foundation and former LLNL associate director of Computation, and Nisha Mulakken, LLNL biostatistician and co-director of the Data Science Summer Institute. Featured speakers will be Amanda Randles, assistant professor at Duke University and former Lawrence Fellow, and LLNL design physicist Kelli Humbird, who is part of the team that predicted the fusion ignition shot at the National Ignition Facility (see seminar recap below).
WiDS Livermore is sponsored by the DSI and LLNL’s Office of Strategic Diversity and Inclusion Programs. Visit the WiDS Livermore web page for all relevant materials: a detailed agenda, virtual backgrounds, directions to LVOC, speed mentoring prompts, and WebEx links.
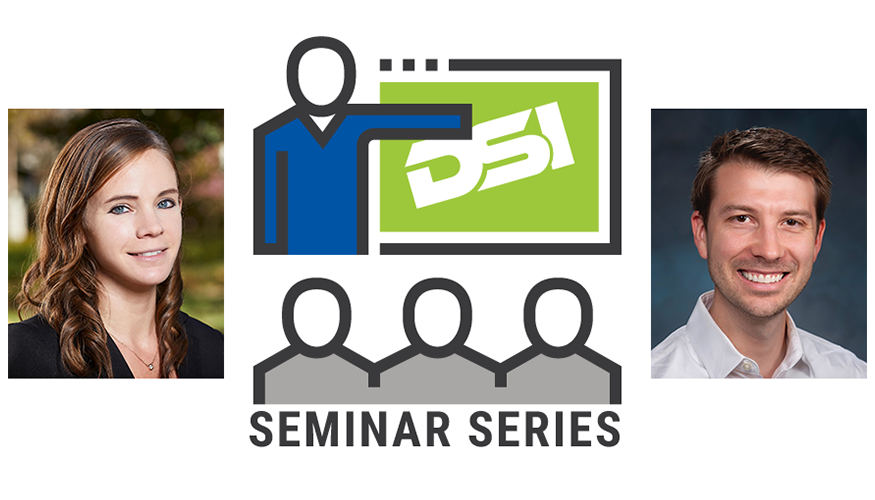
Seminar Reveals the AI Predictions Behind Fusion Ignition
Nearly 400 people attended the DSI’s February seminar, which featured two speakers. LLNL researchers Kelli Humbird and J. Luc Peterson presented “Calling the Shot: How AI Predicted Fusion Ignition Before It Happened.” After several decades of research, the fusion breakthrough at the National Ignition Facility (NIF) brings humanity one step closer to a future of limitless carbon-free, safe, and clean energy. Yet, the shot that finally ushered in the Fusion Age was not actually that surprising. A few hours before the experiment, an LLNL physics team used an artificial intelligence (AI) model to predict the outcome of the experiment. The model, which blends supercomputer simulations with experimental data, indicated that ignition was the most likely outcome for this shot. As such, hopes were high that something big was about to occur. The seminar discussed the breakthrough experiment, nuclear fusion, and how machine learning (ML) was used to call the shot heard around the world.
Dr. Kelli Humbird’s work focuses on ML discovery and design for inertial confinement fusion and integrated hohlraum design. During her time at the Lab, she has worked in stockpile certification, technical nuclear forensics, ML accelerators for multiphysics codes, and ML analysis for the spread of COVID-19 during the first year of the pandemic. The common thread throughout much of her work is the application of ML to scientific problems with sparse data.
Dr. Jayson “Luc” Peterson is the Associate Program Leader for Data Science within LLNL’s Space Science and Security Program, where he is responsible for the leadership and development of a broad portfolio of projects at the intersection of data science and outer space. He also leads the ICECap and Driving Design with Cognitive Simulation projects, which aim to bring ML-enhanced digital design to exascale supercomputers.
The March 14 seminar will feature Dr. Amanda Randles, a former Lawrence Fellow now at Duke University. Speakers’ abstracts are available on the seminar series web page, and many recordings are posted to the YouTube playlist. To become or recommend a speaker for a future seminar, or to request a WebEx link for an upcoming seminar if you’re outside LLNL, contact datascience [at] llnl.gov (datascience[at]llnl[dot]gov).
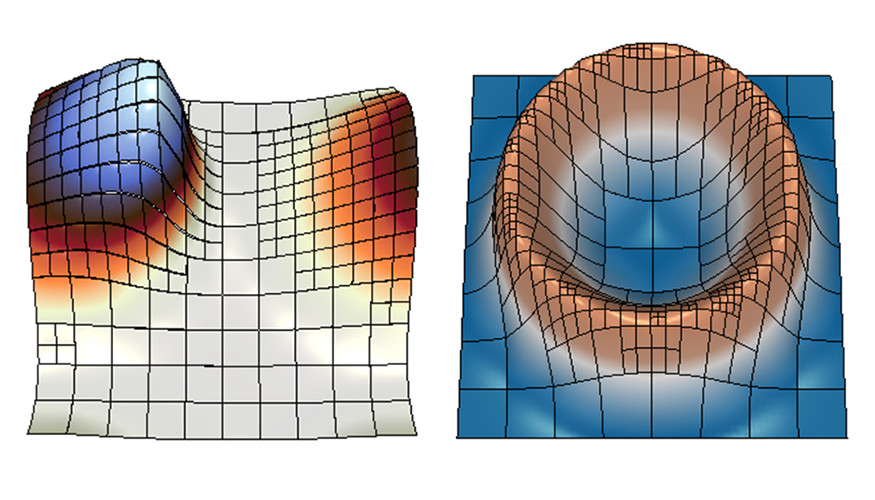
Conference Roundup
LLNL’s data science researchers have had papers accepted at several recent and upcoming conferences that recognize ground-breaking research and techniques. PDFs or abstracts are linked where available.
IEEE/CVF Winter Conference on Applications of Computer Vision (WACV):
- Contrastive knowledge-augmented meta-learning for few-shot classification – Mark Heimann, T.S. Jayram, Rushil Anirudh, Jayaraman Thiagarajan, and an Arizona State University colleague
- Improving diversity with adversarially learned transformations for domain generalization – Anirudh, Thiagarajan, Bhavya Kailkhura, and Arizona State University colleagues
International Conference on Artificial Intelligence and Statistics (AISTATS):
- Reinforcement learning for adaptive mesh refinement – Jiachen Yang, Brenden Petersen, Jun Kudo, Ketan Mittal, Vladimir Tomov, Jean-Sylvain Camier, Tzanio Kolev, Robert Anderson, Daniel Faissol, and colleagues from Texas A&M University and Georgia Institute of Technology (images at left show results of refinement policies trained with reinforcement learning)
International Conference on Autonomous Agents and Multiagent Systems (AAMAS):
- Multi-agent reinforcement learning for adaptive mesh refinement – Yang, Mittal, Socratis Petrides, Brenden Petersen, Faissol, Anderson, and colleagues from Texas A&M University and Brown University
International Conference on Learning Representations (ICLR):
- A closer look at model adaptation using feature distortion and simplicity bias – Thiagarajan and University of Michigan colleagues

Open-Source Software Aids Collaborative Autonomy Applications
The U.S. modern critical infrastructure—from the electrical grid that sends power to homes to the pipelines that deliver water and natural gas and the railways and roadways we travel—is full of digitized components. In a power grid, this includes distributed energy resources such as smart meters, solar inverters, power-quality sensors, and protection devices that are geographically spread out, programmable and network connected. These networks, as currently designed, typically rely on a single control center for analysis and decision making.
To defend against cyber-attacks and harden the system, LLNL mathematicians, systems analysts, power engineers, cybersecurity experts and computational scientists have turned to collaborative autonomy—a new class of computational techniques that teach networked devices how to self-organize into a collective whole. And recently, an LLNL research team has developed Skywing—an open-source, high-reliability, real-time, decentralized software platform for domain scientists, mathematicians, and computer scientists exploring collaborative-autonomy applications for critical infrastructure. Skywing provides approaches and solutions for real-world applications that solve problems and allow for confidence in the results. It also helps lower the barrier to entry for those who may lack fluency in decentralized software development. Skywing is available on GitHub.
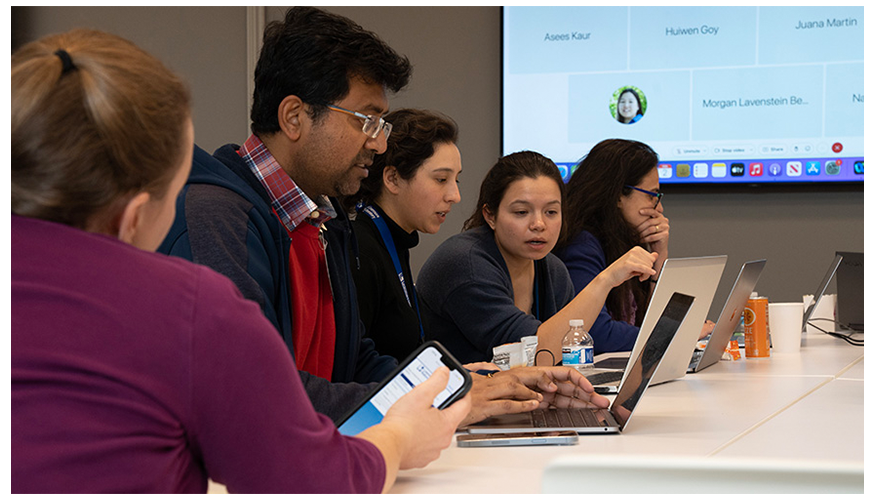
Hackathon Highlights Employee-Student Collaboration and Women in Data Science
The DSI sponsored a combined hackathon/datathon on February 2–3. Organizers invited students from local universities and high schools to team up with Lab scientists to network, brainstorm, and code. The event featured a special WiDS climate dataset challenge for teams to work on.
Held at the Lab’s new LVOC facility, the 24-hour event provided attendees both online and in-person the opportunity to try out new data science technologies and work with colleagues and students on various projects, some of them carried over from previous Lab hackathons. In true hackathon tradition, many participants chose to work through the night, with teams presenting their projects the following day.
The event also included a keynote presentation by University of California (UC) Merced applied math professor Suzanne Sindi and talks by Climate LLC data scientist Kelsey Blackstone and LLNL climate physics researcher Gemma Anderson. Blackstone spoke about ethical considerations in statistical modeling and her research applying data analytics to agriculture, while Anderson discussed how artificial intelligence is being used to improve the resolution and accuracy of climate predictions.
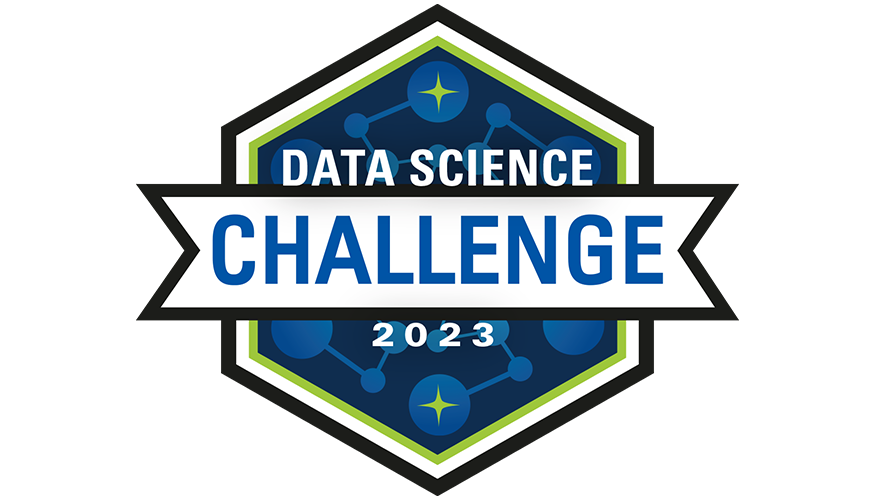
Preview of the Data Science Challenge Combined Session
The Data Science Challenge (DSC) returns in July to provide students from UC Merced and UC Riverside with a two-week, intensive internship at the Lab’s UC Livermore Collaboration Center (UCLCC). Undergraduate and graduate students will work together on a challenge problem in cardiology, where they will explore a data-driven approach to reconstructing electro-anatomical maps of the heart at clinically relevant resolutions, combining input from the standard 12-lead electrocardiogram with advanced machine learning techniques.
LLNL computer scientist Brian Gallagher and data scientist Cindy Gonzales will mentor the students alongside a professor from each university. “We have a number of exciting improvements to DSC we are looking forward to this year. Thanks to support from UCLCC, DSC will be back in person in Livermore after several years of remote and UC-hosted programs,” Gallagher says. “We will also be holding a joint program with UC Merced and UC Riverside together for the first time ever. And last, but not least, I am very pleased to welcome Cindy Gonzales as my LLNL co-director this year.” Read about previous DSC sessions on the DSI website.

Meet an LLNL Data Scientist
Working at LLNL gives Ruben Glatt, a senior staff researcher in machine learning, the opportunity to solve globally important problems. He currently leads a Laboratory Directed Research and Development feasibility study using reinforcement learning (RL) to investigate energy-efficient transportation and, in other projects, applies generative models and domain concepts to improve trust in model predictions. “Keeping up with the developments in the fields of data science and machine learning can pose a challenge, yet the recent progress made in these areas holds immense potential to revolutionize energy generation, healthcare, and other industries,” he explains. “As we embark on a new era where artificial intelligence holds the power to master an increasing number of processes, it is my aspiration to ensure that research remains aligned with humanistic values, so that we can not only fully realize the benefits of these advancements, but also minimize any potential existential risks.” In 2022, Glatt chaired the Lab’s Center for Advanced Signal and Image Sciences (CASIS) workshop and was part of the research team whose deep symbolic optimization method won the first-ever worldwide symbolic regression competition. He has presented his research at numerous venues including premier machine learning conferences, and contributed a chapter on efficient RL to the Federated and Transfer Learning book, published by Springer in 2022. Glatt joined the Lab in 2019 after completing a PhD in Computer Engineering with a focus on knowledge transfer in RL.